Intraoperative Fluorescence Imaging for Personalized Brain Tumor Resection: Current State and Future Directions
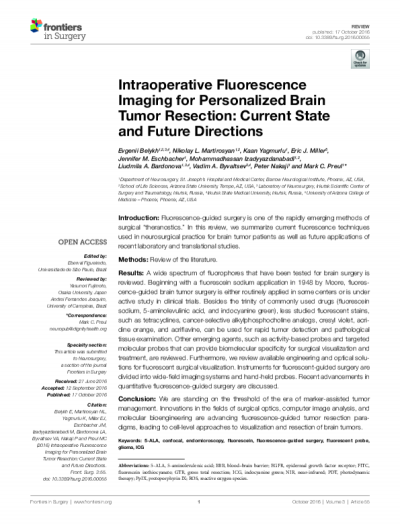
Introduction: Fluorescence-guided surgery is one of the rapidly emerging methods of surgical “theranostics.” In this review, we summarize current fluorescence techniques used in neurosurgical practice for brain tumor patients as well as future applications of recent laboratory and translational studies.
Methods: Review of the literature.
Results: A wide spectrum of fluorophores that have been tested for brain surgery is reviewed. Beginning with a fluorescein sodium application in 1948 by Moore, fluorescence-guided brain tumor surgery is either routinely applied in some centers or is under active study in clinical trials. Besides the trinity of commonly used drugs (fluorescein sodium, 5-aminolevulinic acid, and indocyanine green), less studied fluorescent stains, such as tetracyclines, cancer-selective alkylphosphocholine analogs, cresyl violet, acridine orange, and acriflavine, can be used for rapid tumor detection and pathological tissue examination. Other emerging agents, such as activity-based probes and targeted molecular probes that can provide biomolecular specificity for surgical visualization and treatment, are reviewed. Furthermore, we review available engineering and optical solutions for fluorescent surgical visualization. Instruments for fluorescent-guided surgery are divided into wide-field imaging systems and hand-held probes. Recent advancements in quantitative fluorescence-guided surgery are discussed.
Conclusion: We are standing on the threshold of the era of marker-assisted tumor management. Innovations in the fields of surgical optics, computer image analysis, and molecular bioengineering are advancing fluorescence-guided tumor resection paradigms, leading to cell-level approaches to visualization and resection of brain tumors.
- Author (aut): Belykh, Evgenii
- Author (aut): Martirosyan, Nikolay
- Author (aut): Yagmurlu, Kaan
- Author (aut): Miller, Eric J.
- Author (aut): Eschbacher, Jennifer M.
- Author (aut): Izady Yazdanabadi, Mohammadhassan
- Author (aut): Bardonova, Liudmila A.
- Author (aut): Byvaltsev, Vadim A.
- Author (aut): Nakaji, Peter
- Author (aut): Preul, Mark C.
- Contributor (ctb): College of Liberal Arts and Sciences