Texture analysis platform for imaging biomarker research
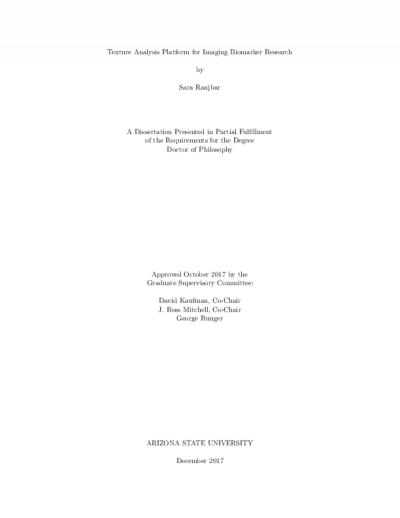
Because of its established role in cancer care and its non-invasive nature imaging offers the potential to complement the findings of cancer biology. Over the past decade, a compelling body of literature has emerged suggesting a more pivotal role for imaging in the diagnosis, prognosis, and monitoring of diseases. These advances have facilitated the rise of an emerging practice known as Radiomics: the extraction and analysis of large numbers of quantitative features from medical images to improve disease characterization and prediction of outcome. It has been suggested that radiomics can contribute to biomarker discovery by detecting imaging traits that are complementary or interchangeable with other markers.
This thesis seeks further advancement of imaging biomarker discovery. This research unfolds over two aims: I) developing a comprehensive methodological pipeline for converting diagnostic imaging data into mineable sources of information, and II) investigating the utility of imaging data in clinical diagnostic applications. Four validation studies were conducted using the radiomics pipeline developed in aim I. These studies had the following goals: (1 distinguishing between benign and malignant head and neck lesions (2) differentiating benign and malignant breast cancers, (3) predicting the status of Human Papillomavirus in head and neck cancers, and (4) predicting neuropsychological performances as they relate to Alzheimer’s disease progression. The long-term objective of this thesis is to improve patient outcome and survival by facilitating incorporation of routine care imaging data into decision making processes.
- Author (aut): Ranjbar, Sara
- Thesis advisor (ths): Kaufman, David
- Thesis advisor (ths): Mitchell, Joseph R.
- Committee member: Runger, George C.
- Publisher (pbl): Arizona State University