Data Fusion and Systems Engineering Approaches for Quality and Performance Improvement of Health Care Systems: From Diagnosis to Care to System-level Decision-making
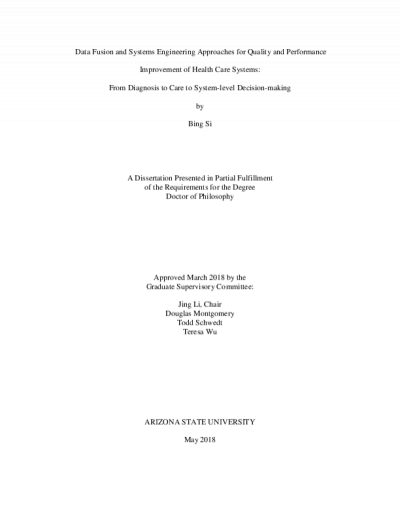
The first topic is diagnosis/subtyping of migraine to customize effective treatment to different subtypes of patients. Existing clinical definitions of subtypes use somewhat arbitrary boundaries primarily based on patient self-reported symptoms, which are subjective and error-prone. My research develops a novel Multimodality Factor Mixture Model that discovers subtypes of migraine from multimodality imaging MRI data, which provides complementary accurate measurements of the disease. Patients in the different subtypes show significantly different clinical characteristics of the disease. Treatment tailored and optimized for patients of the same subtype paves the road toward Precision Medicine.
The second topic focuses on coordinated patient care. Care coordination between nurses and with other health care team members is important for providing high-quality and efficient care to patients. The recently developed Nurse Care Coordination Instrument (NCCI) is the first of its kind that enables large-scale quantitative data to be collected. My research develops a novel Multi-response Multi-level Model (M3) that enables transfer learning in NCCI data fusion. M3 identifies key factors that contribute to improving care coordination, and facilitates the design and optimization of nurses’ training, workload assignment, and practice environment, which leads to improved patient outcomes.
The last topic is about system-level decision-making for Alzheimer’s disease early detection at the early stage of Mild Cognitive Impairment (MCI), by predicting each MCI patient’s risk of converting to AD using imaging and proteomic biomarkers. My research proposes a systems engineering approach that integrates the multi-perspectives, including prediction accuracy, biomarker cost/availability, patient heterogeneity and diagnostic efficiency, and allows for system-wide optimized decision regarding the biomarker testing process for prediction of MCI conversion.
- Author (aut): Si, Bing
- Thesis advisor (ths): Li, Jing
- Committee member: Montgomery, Douglas C.
- Committee member: Schwedt, Todd
- Committee member: Wu, Teresa
- Publisher (pbl): Arizona State University