Improving solar PV scheduling using statistical techniques
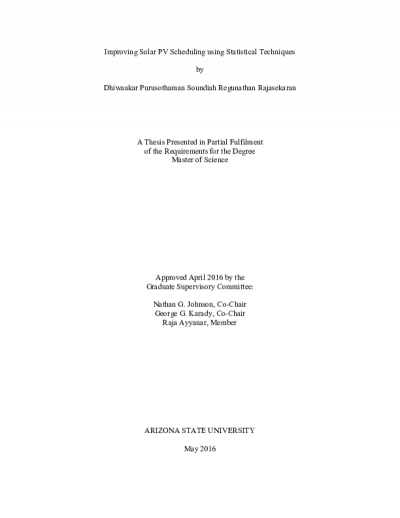
Description
The inherent intermittency in solar energy resources poses challenges to scheduling generation, transmission, and distribution systems. Energy storage devices are often used to mitigate variability in renewable asset generation and provide a mechanism to shift renewable power between periods of the day. In the absence of storage, however, time series forecasting techniques can be used to estimate future solar resource availability to improve the accuracy of solar generator scheduling. The knowledge of future solar availability helps scheduling solar generation at high-penetration levels, and assists with the selection and scheduling of spinning reserves. This study employs statistical techniques to improve the accuracy of solar resource forecasts that are in turn used to estimate solar photovoltaic (PV) power generation. The first part of the study involves time series forecasting of the global horizontal irradiation (GHI) in Phoenix, Arizona using Seasonal Autoregressive Integrated Moving Average (SARIMA) models. A comparative study is completed for time series forecasting models developed with different time step resolutions, forecasting start time, forecasting time horizons, training data, and transformations for data measured at Phoenix, Arizona. Approximately 3,000 models were generated and evaluated across the entire study. One major finding is that forecasted values one day ahead are near repeats of the preceding day—due to the 24-hour seasonal differencing—indicating that use of statistical forecasting over multiple days creates a repeating pattern. Logarithmic transform data were found to perform poorly in nearly all cases relative to untransformed or square-root transform data when forecasting out to four days. Forecasts using a logarithmic transform followed a similar profile as the immediate day prior whereas forecasts using untransformed and square-root transform data had smoother daily solar profiles that better represented the average intraday profile. Error values were generally lower during mornings and evenings and higher during midday. Regarding one-day forecasting and shorter forecasting horizons, the logarithmic transformation performed better than untransformed data and square-root transformed data irrespective of forecast horizon for data resolutions of 1-hour, 30-minutes, and 15-minutes.
Date Created
The date the item was original created (prior to any relationship with the ASU Digital Repositories.)
2016
Agent
- Author (aut): Soundiah Regunathan Rajasekaran, Dhiwaakar Purusothaman
- Thesis advisor (ths): Johnson, Nathan G
- Thesis advisor (ths): Karady, George G.
- Committee member: Ayyanar, Raja
- Publisher (pbl): Arizona State University