Investigating the Role of Silent Users on Social Media
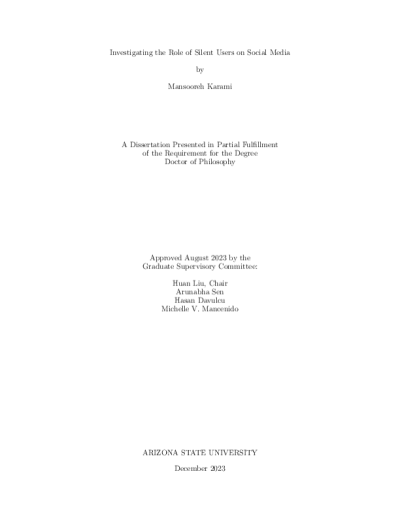
Description
Social media platforms provide a rich environment for analyzing user behavior. Recently, deep learning-based methods have been a mainstream approach for social media analysis models involving complex patterns. However, these methods are susceptible to biases in the training data, such as participation inequality. Basically, a mere 1% of users generate the majority of the content on social networking sites, while the remaining users, though engaged to varying degrees, tend to be less active in content creation and largely silent. These silent users consume and listen to information that is propagated on the platform.However, their voice, attitude, and interests are not reflected in the online content, making the decision of the current methods predisposed towards the opinion of the active users. So models can mistake the loudest users for the majority. To make the silent majority heard is to reveal the true landscape of the platform. In this dissertation, to compensate for this bias in the data, which is related to user-level data scarcity, I introduce three pieces of research work. Two of these proposed solutions deal with the data on hand while the other tries to augment the current data. Specifically, the first proposed approach modifies the weight of users' activity/interaction in the input space, while the second approach involves re-weighting the loss based on the users' activity levels during the downstream task training. Lastly, the third approach uses large language models (LLMs) and learns the user's writing behavior to expand the current data. In other words, by utilizing LLMs as a sophisticated knowledge base, this method aims to augment the silent user's data.
Date Created
The date the item was original created (prior to any relationship with the ASU Digital Repositories.)
2023
Agent
- Author (aut): Karami, Mansooreh
- Thesis advisor (ths): Liu, Huan
- Committee member: Sen, Arunabha
- Committee member: Davulcu, Hasan
- Committee member: Mancenido, Michelle V.
- Publisher (pbl): Arizona State University