Oxygen Ionic-Conducting Ceramics for Gas Separation and Reaction Applications
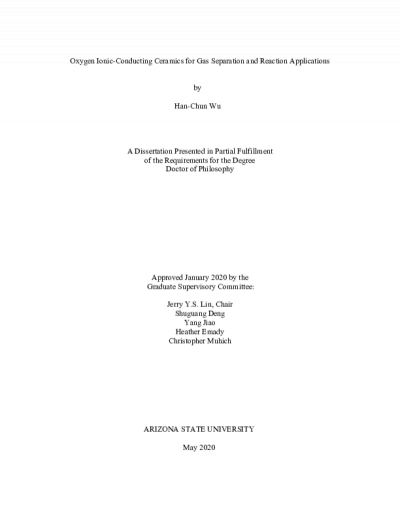
Description
Mixed-ionic electronic conducting (MIEC) oxides have drawn much attention from researchers because of their potential in high temperature separation processes. Among many materials available, perovskite type and fluorite type oxides are the most studied for their excellent oxygen ion transport property. These oxides not only can be oxygen adsorbent or O2-permeable membranes themselves, but also can be incorporated with molten carbonate to form dual-phase membranes for CO2 separation.
Oxygen sorption/desorption properties of perovskite oxides with and without oxygen vacancy were investigated first by thermogravimetric analysis (TGA) and fixed-bed experiments. The oxide with unique disorder-order phase transition during desorption exhibited an enhanced oxygen desorption rate during the TGA measurement but not in fixed-bed demonstrations. The difference in oxygen desorption rate is due to much higher oxygen partial pressure surrounding the sorbent during the fixed-bed oxygen desorption process, as revealed by X-ray diffraction (XRD) patterns of rapidly quenched samples.
Research on using perovskite oxides as CO2-permeable dual-phase membranes was subsequently conducted. Two CO2-resistant MIEC perovskite ceramics, Pr0.6Sr0.4Co0.2Fe0.8 O3-δ (PSCF) and SrFe0.9Ta0.1O3-δ (SFT) were chosen as support materials for membrane synthesis. PSCF-molten carbonate (MC) and SFT-MC membranes were prepared for CO2-O2 counter-permeation. The geometric factors for the carbonate phase and ceramic phase were used to calculate the effective carbonate and oxygen ionic conductivity in the carbonate and ceramic phase. When tested in CO2-O2 counter-permeation set-up, CO2 flux showed negligible change, but O2 flux decreased by 10-32% compared with single-component permeation. With CO2 counter-permeation, the total oxygen permeation flux is higher than that without counter-permeation.
A new concept of CO2-permselective membrane reactor for hydrogen production via steam reforming of methane (SRM) was demonstrated. The results of SRM in the membrane reactor confirm that in-situ CO2 removal effectively promotes water-gas shift conversion and thus enhances hydrogen yield. A modeling study was also conducted to assess the performance of the membrane reactor in high-pressure feed/vacuum sweep conditions, which were not carried out due to limitations in current membrane testing set-up. When 5 atm feed pressure and 10-3 atm sweep pressure were applied, the membrane reactor can produce over 99% hydrogen stream in simulation.
Oxygen sorption/desorption properties of perovskite oxides with and without oxygen vacancy were investigated first by thermogravimetric analysis (TGA) and fixed-bed experiments. The oxide with unique disorder-order phase transition during desorption exhibited an enhanced oxygen desorption rate during the TGA measurement but not in fixed-bed demonstrations. The difference in oxygen desorption rate is due to much higher oxygen partial pressure surrounding the sorbent during the fixed-bed oxygen desorption process, as revealed by X-ray diffraction (XRD) patterns of rapidly quenched samples.
Research on using perovskite oxides as CO2-permeable dual-phase membranes was subsequently conducted. Two CO2-resistant MIEC perovskite ceramics, Pr0.6Sr0.4Co0.2Fe0.8 O3-δ (PSCF) and SrFe0.9Ta0.1O3-δ (SFT) were chosen as support materials for membrane synthesis. PSCF-molten carbonate (MC) and SFT-MC membranes were prepared for CO2-O2 counter-permeation. The geometric factors for the carbonate phase and ceramic phase were used to calculate the effective carbonate and oxygen ionic conductivity in the carbonate and ceramic phase. When tested in CO2-O2 counter-permeation set-up, CO2 flux showed negligible change, but O2 flux decreased by 10-32% compared with single-component permeation. With CO2 counter-permeation, the total oxygen permeation flux is higher than that without counter-permeation.
A new concept of CO2-permselective membrane reactor for hydrogen production via steam reforming of methane (SRM) was demonstrated. The results of SRM in the membrane reactor confirm that in-situ CO2 removal effectively promotes water-gas shift conversion and thus enhances hydrogen yield. A modeling study was also conducted to assess the performance of the membrane reactor in high-pressure feed/vacuum sweep conditions, which were not carried out due to limitations in current membrane testing set-up. When 5 atm feed pressure and 10-3 atm sweep pressure were applied, the membrane reactor can produce over 99% hydrogen stream in simulation.
Date Created
The date the item was original created (prior to any relationship with the ASU Digital Repositories.)
2020
Agent
- Author (aut): Wu, Han-Chun
- Thesis advisor (ths): Lin, Jerry Y.S.
- Committee member: Deng, Shuguang
- Committee member: Jiao, Yang
- Committee member: Emady, Heather
- Committee member: Muhich, Christopherq
- Publisher (pbl): Arizona State University