Bayesian Hierarchical Model for Testing Allele Specific Expression Towards the Alternative Allele
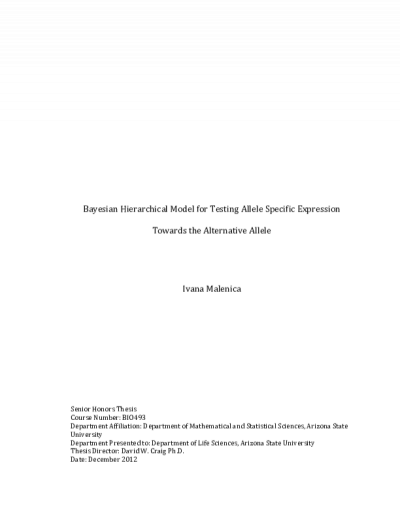
Description
Identifying associations between genotypes and gene expression levels using next-generation technology has enabled systematic interrogation of regulatory variation underlying complex phenotypes. Understanding the source of expression variation has important implications for disease susceptibility, phenotypic diversity, and adaptation (Main, 2009). Interest in the existence of allele-specific expression in autosomal genes evolved with the increased awareness of the important role that variation in non-coding DNA sequences can play in determining phenotypic diversity, and the essential role parent-of-origin expression has in early development (Knight, 2004). As new implications of high-throughput sequencing are conceived, it is becoming increasingly important to develop statistical methods tailored to large and formidably complex data sets in order to maximize the biological insights derived from next-generation sequencing experiments. Here, a Bayesian hierarchical probability model based on the beta-binomial distribution is proposed as a possible approach for quantifying allele-specific expression from whole genome (WGS) and whole transcriptome (RNA-seq) data. Pipeline for the analysis of WGS and RNA-seq data sets from ten samples was developed and implemented, while allele-specific expression (ASE) was quantified from both haplotypes using individuals heterozygous at the tested variants utilizing the described methodology. Both computational and statistical framework applied accurately quantified ASE, achieving high reproducibility of already described allele-specific genes in the literature. In conclusion, described methodology provides a solid starting point for quantifying allele specific expression across whole genomes.
Date Created
The date the item was original created (prior to any relationship with the ASU Digital Repositories.)
2012-12
Agent
- Author (aut): Malenica, Ivana
- Thesis director: Craig, David
- Committee member: Rosenberg, Michael
- Committee member: Szelinger, Szabolcs
- Contributor (ctb): Barrett, The Honors College
- Contributor (ctb): College of Liberal Arts and Sciences