Analysis and Management of Security State for Large-Scale Data Center Networks
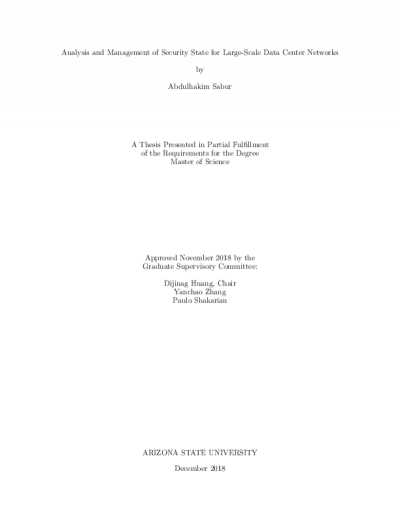
Description
With the increasing complexity of computing systems and the rise in the number of risks and vulnerabilities, it is necessary to provide a scalable security situation awareness tool to assist the system administrator in protecting the critical assets, as well as managing the security state of the system. There are many methods to provide security states' analysis and management. For instance, by using a Firewall to manage the security state, and/or a graphical analysis tools such as attack graphs for analysis.
Attack Graphs are powerful graphical security analysis tools as they provide a visual representation of all possible attack scenarios that an attacker may take to exploit system vulnerabilities. The attack graph's scalability, however, is a major concern for enumerating all possible attack scenarios as it is considered an NP-complete problem. There have been many research work trying to come up with a scalable solution for the attack graph. Nevertheless, non-practical attack graph based solutions have been used in practice for realtime security analysis.
In this thesis, a new framework, namely 3S (Scalable Security Sates) analysis framework is proposed, which present a new approach of utilizing Software-Defined Networking (SDN)-based distributed firewall capabilities and the concept of stateful data plane to construct scalable attack graphs in near-realtime, which is a practical approach to use attack graph for realtime security decisions. The goal of the proposed work is to control reachability information between different datacenter segments to reduce the dependencies among vulnerabilities and restrict the attack graph analysis in a relative small scope. The proposed framework is based on SDN's programmable capabilities to adjust the distributed firewall policies dynamically according to security situations during the running time. It apply white-list-based security policies to limit the attacker's capability from moving or exploiting different segments by only allowing uni-directional vulnerability dependency links between segments. Specifically, several test cases will be presented with various attack scenarios and analyze how distributed firewall and stateful SDN data plan can significantly reduce the security states construction and analysis. The proposed approach proved to achieve a percentage of improvement over 61% in comparison with prior modules were SDN and distributed firewall are not in use.
Attack Graphs are powerful graphical security analysis tools as they provide a visual representation of all possible attack scenarios that an attacker may take to exploit system vulnerabilities. The attack graph's scalability, however, is a major concern for enumerating all possible attack scenarios as it is considered an NP-complete problem. There have been many research work trying to come up with a scalable solution for the attack graph. Nevertheless, non-practical attack graph based solutions have been used in practice for realtime security analysis.
In this thesis, a new framework, namely 3S (Scalable Security Sates) analysis framework is proposed, which present a new approach of utilizing Software-Defined Networking (SDN)-based distributed firewall capabilities and the concept of stateful data plane to construct scalable attack graphs in near-realtime, which is a practical approach to use attack graph for realtime security decisions. The goal of the proposed work is to control reachability information between different datacenter segments to reduce the dependencies among vulnerabilities and restrict the attack graph analysis in a relative small scope. The proposed framework is based on SDN's programmable capabilities to adjust the distributed firewall policies dynamically according to security situations during the running time. It apply white-list-based security policies to limit the attacker's capability from moving or exploiting different segments by only allowing uni-directional vulnerability dependency links between segments. Specifically, several test cases will be presented with various attack scenarios and analyze how distributed firewall and stateful SDN data plan can significantly reduce the security states construction and analysis. The proposed approach proved to achieve a percentage of improvement over 61% in comparison with prior modules were SDN and distributed firewall are not in use.
Date Created
The date the item was original created (prior to any relationship with the ASU Digital Repositories.)
2018
Agent
- Author (aut): Sabur, Abdulhakim
- Thesis advisor (ths): Huang, Dijiang
- Committee member: Zhang, Yancho
- Committee member: Shakarian, Paulo
- Publisher (pbl): Arizona State University