GPU-enabled Functional-as-a-Service
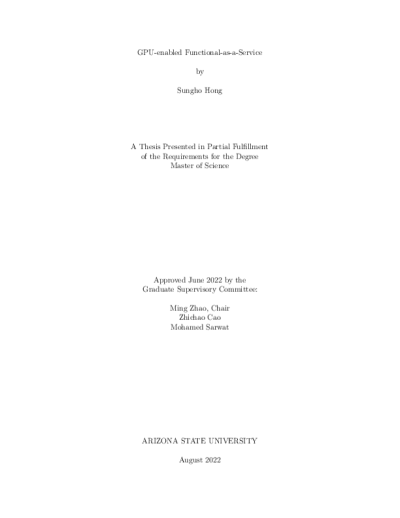
- Author (aut): Hong, Sungho
- Thesis advisor (ths): Zhao, Ming
- Committee member: Cao, Zhichao
- Committee member: Sarwat, Mohamed
- Publisher (pbl): Arizona State University
Data integration involves the reconciliation of data from diverse data sources in order to obtain a unified data repository, upon which an end user such as a data analyst can run analytics sessions to explore the data and obtain useful insights. Supervised Machine Learning (ML) for data integration tasks such as ontology (schema) or entity (instance) matching requires several training examples in terms of manually curated, pre-labeled matching and non-matching schema concept or entity pairs which are hard to obtain. On similar lines, an analytics system without predictive capabilities about the impending workload can incur huge querying latencies, while leaving the onus of understanding the underlying database schema and writing a meaningful query at every step during a data exploration session on the user. In this dissertation, I will describe the human-in-the-loop Machine Learning (ML) systems that I have built towards data integration and predictive analytics. I alleviate the need for extensive prior labeling by utilizing active learning (AL) for dataintegration. In each AL iteration, I detect the unlabeled entity or schema concept pairs that would strengthen the ML classifier and selectively query the human oracle for such labels in a budgeted fashion. Thus, I make use of human assistance for ML-based data integration. On the other hand, when the human is an end user exploring data through Online Analytical Processing (OLAP) queries, my goal is to pro-actively assist the human by predicting the top-K next queries that s/he is likely to be interested in. I will describe my proposed SQL-predictor, a Business Intelligence (BI) query predictor and a geospatial query cardinality estimator with an emphasis on schema abstraction, query representation and how I adapt the ML models for these tasks. For each system, I will discuss the evaluation metrics and how the proposed systems compare to the state-of-the-art baselines on multiple datasets and query workloads.