Building Causal Narratives on Continuous Ensemble Simulation Data
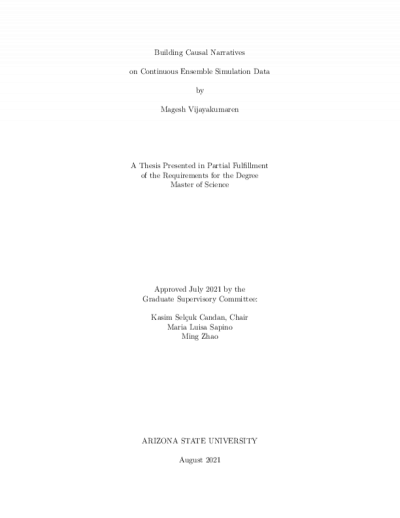
Description
Data-driven simulations represent a promising approach to understanding and predicting complex dynamic processes in the presence of shifting demands of urban systems. Newly proposed continuous ensemble frameworks like DataStorm, execute the models in coupled continuous simulations, thus producing multiple outputs per execution of the model, i.e., any time instant may be covered by multiple simulation ensembles of the corresponding model, each with a different set of data and parameter values. Continuous frameworks focus on designing ensemble configurations that appropriately and efficiently cover the input parameter space, and do not give importance to building causal narratives during continuous execution, which is essentially important for understanding and interpreting the end simulation data. The thesis aims to address this challenge by building causal-fabrics during continuous execution, which essentially contributes to causal-creation of simulation ensembles, which are similar to its previous history, input sample-parameter and output-stream explored. I introduce the following metrics: Provenance-similarity, Output-similarity, and parametric-similarity which could be used to weave such acausal-fabric into explainable causal-narratives. The DataStorm execution framework runs the models in different ensemble configurations to cover the input parameter space efficiently. An end-user may have a preference for a parameter subspace and may seek to find causal narratives where the ensembles in the causal narratives reside in that parameter sub-space. I present an additional constraint called Preference Query E, that defines such a preferred input parameter subspace. I propose a new method of ensemble creation, where during each execution step in continuous execution, more bias is given to the creation of new ensembles that are present in that preferred parameter subspace. Once such narratives have been constructed, I use the concept of timelines to build causal explorations of the causal narratives that have been constructed. I present an approximate top-K timelines algorithm that discovers K such timelines using heuristic search. The next step is to find time-lines that are causal-explorations in the preferred parameter subspace. Using a probabilistic skyline query, I aim to discover a subset of top-K timelines where each timeline has the maximum number of simulation ensembles present in the desired parameter subspace. These subsets of timelines effectively describe such causal exploration in the preferred parameter subspace.
Date Created
The date the item was original created (prior to any relationship with the ASU Digital Repositories.)
2021
Agent
- Author (aut): Vijayakumaren, Magesh
- Thesis advisor (ths): Candan, Selçuk kasim
- Committee member: Zhao, Ming
- Committee member: Sapino, Maria Luisa
- Publisher (pbl): Arizona State University