Implicit Hypothetical Reasoning about Intrinsic Physical Properties
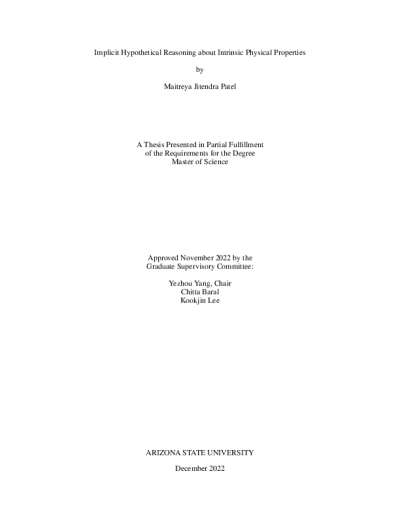
Description
Multimodal reasoning is one of the most interesting research fields because of the ability to interact with systems and the explainability of the models' behavior. Traditional multimodal research problems do not focus on complex commonsense reasoning (such as physical interactions). Although real-world objects have physical properties associated with them, many of these properties (such as mass and coefficient of friction) are not captured directly by the imaging pipeline. Videos often capture objects, their motion, and the interactions between different objects. However, these properties can be estimated by utilizing cues from relative object motion and the dynamics introduced by collisions. This thesis introduces a new video question-answering task for reasoning about the implicit physical properties of objects in a scene, from videos. For this task, I introduce a dataset -- CRIPP-VQA (Counterfactual Reasoning about Implicit Physical Properties - Video Question Answering), which contains videos of objects in motion, annotated with hypothetical/counterfactual questions about the effect of actions (such as removing, adding, or replacing objects), questions about planning (choosing actions to perform to reach a particular goal), as well as descriptive questions about the visible properties of objects. Further, I benchmark the performance of existing video question-answering models on two test settings of CRIPP-VQA: i.i.d. and an out-of-distribution setting which contains objects with values of mass, coefficient of friction, and initial velocities that are not seen in the training distribution. Experiments reveal a surprising and significant performance gap in terms of answering questions about implicit properties (the focus of this thesis) and explicit properties (the focus of prior work) of objects.
Date Created
The date the item was original created (prior to any relationship with the ASU Digital Repositories.)
2022
Agent
- Author (aut): Patel, Maitreya Jitendra
- Thesis advisor (ths): Yang, Yezhou
- Committee member: Baral, Chitta
- Committee member: Lee, Kookjin
- Publisher (pbl): Arizona State University