QU-Net: A Lightweight U-Net based Region Proposal System
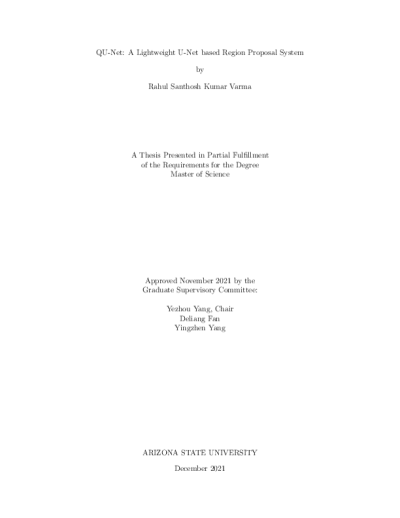
- Author (aut): Santhosh Kumar Varma, Rahul
- Thesis advisor (ths): Yang, Yezhou
- Committee member: Fan, Deliang
- Committee member: Yang, Yingzhen
- Publisher (pbl): Arizona State University
Molecular pathology makes use of estimates of tumor content (tumor percentage) for pre-analytic and analytic purposes, such as molecular oncology testing, massive parallel sequencing, or next-generation sequencing (NGS), assessment of sample acceptability, accurate quantitation of variants, assessment of copy number changes (among other applications), determination of specimen viability for testing (since many assays require a minimum tumor content to report variants at the limit of detection) may all be improved with more accurate and reproducible estimates of tumor content. Currently, tumor percentages of samples submitted for molecular testing are estimated by visual examination of Hematoxylin and Eosin (H&E) stained tissue slides under the microscope by pathologists. These estimations can be automated, expedited, and rendered more accurate by applying machine learning methods on digital whole slide images (WSI).