Producing Acoustic-Prosodic Entrainment in a Robotic Learning Companion to Build Learner Rapport
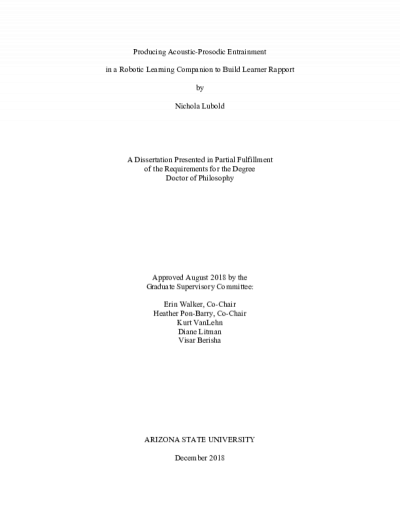
Description
With advances in automatic speech recognition, spoken dialogue systems are assuming increasingly social roles. There is a growing need for these systems to be socially responsive, capable of building rapport with users. In human-human interactions, rapport is critical to patient-doctor communication, conflict resolution, educational interactions, and social engagement. Rapport between people promotes successful collaboration, motivation, and task success. Dialogue systems which can build rapport with their user may produce similar effects, personalizing interactions to create better outcomes.
This dissertation focuses on how dialogue systems can build rapport utilizing acoustic-prosodic entrainment. Acoustic-prosodic entrainment occurs when individuals adapt their acoustic-prosodic features of speech, such as tone of voice or loudness, to one another over the course of a conversation. Correlated with liking and task success, a dialogue system which entrains may enhance rapport. Entrainment, however, is very challenging to model. People entrain on different features in many ways and how to design entrainment to build rapport is unclear. The first goal of this dissertation is to explore how acoustic-prosodic entrainment can be modeled to build rapport.
Towards this goal, this work presents a series of studies comparing, evaluating, and iterating on the design of entrainment, motivated and informed by human-human dialogue. These models of entrainment are implemented in the dialogue system of a robotic learning companion. Learning companions are educational agents that engage students socially to increase motivation and facilitate learning. As a learning companion’s ability to be socially responsive increases, so do vital learning outcomes. A second goal of this dissertation is to explore the effects of entrainment on concrete outcomes such as learning in interactions with robotic learning companions.
This dissertation results in contributions both technical and theoretical. Technical contributions include a robust and modular dialogue system capable of producing prosodic entrainment and other socially-responsive behavior. One of the first systems of its kind, the results demonstrate that an entraining, social learning companion can positively build rapport and increase learning. This dissertation provides support for exploring phenomena like entrainment to enhance factors such as rapport and learning and provides a platform with which to explore these phenomena in future work.
This dissertation focuses on how dialogue systems can build rapport utilizing acoustic-prosodic entrainment. Acoustic-prosodic entrainment occurs when individuals adapt their acoustic-prosodic features of speech, such as tone of voice or loudness, to one another over the course of a conversation. Correlated with liking and task success, a dialogue system which entrains may enhance rapport. Entrainment, however, is very challenging to model. People entrain on different features in many ways and how to design entrainment to build rapport is unclear. The first goal of this dissertation is to explore how acoustic-prosodic entrainment can be modeled to build rapport.
Towards this goal, this work presents a series of studies comparing, evaluating, and iterating on the design of entrainment, motivated and informed by human-human dialogue. These models of entrainment are implemented in the dialogue system of a robotic learning companion. Learning companions are educational agents that engage students socially to increase motivation and facilitate learning. As a learning companion’s ability to be socially responsive increases, so do vital learning outcomes. A second goal of this dissertation is to explore the effects of entrainment on concrete outcomes such as learning in interactions with robotic learning companions.
This dissertation results in contributions both technical and theoretical. Technical contributions include a robust and modular dialogue system capable of producing prosodic entrainment and other socially-responsive behavior. One of the first systems of its kind, the results demonstrate that an entraining, social learning companion can positively build rapport and increase learning. This dissertation provides support for exploring phenomena like entrainment to enhance factors such as rapport and learning and provides a platform with which to explore these phenomena in future work.
Date Created
The date the item was original created (prior to any relationship with the ASU Digital Repositories.)
2018
Agent
- Author (aut): Lubold, Nichola Anne
- Thesis advisor (ths): Walker, Erin
- Thesis advisor (ths): Pon-Barry, Heather
- Committee member: Litman, Diane
- Committee member: VanLehn, Kurt
- Committee member: Berisha, Visar
- Publisher (pbl): Arizona State University