Robust Performance Monitoring for Adaptive PID Controllers in System Recovery from Insufficient Excitation
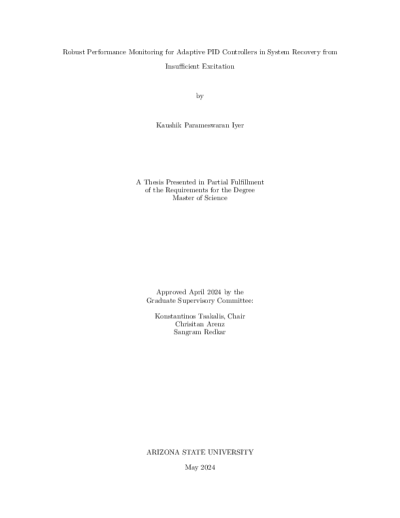
Description
The aim of this thesis is to study adaptive controllers in the context of a Pro-portional Integral Derivative (PID) controller. The PID controller is tuned via loop shaping techniques to ensure desired robustness and performance characteristics with respect to a target loop shape. There are two problems that this work addresses: Consider a system that is controlled via an adaptive PID controller. If in absence of or under lack of excitation, the system or controller parameters drift to an arbitrary system (that may or may not be stable). Then, once the system gets sufficient ex- citation, there are two questions to be addressed: First, how quickly is the system able to recover to the target system, and in the process of recovery, how large are the transient overshoots and what factors affect the recovery of the drifted system? Second, continuous online adaptation of the controller may not always be necessary (and economical). So, is there a means to monitor the performance of the current controller and determine via robustness conditions whether to continue with the same controller or reject it and adapt to a new controller? Hence, this work is concerned with robust performance monitoring and recovery of an adaptive PID control system that had drifted to another system in absence of sufficient excitation or excessive noise.
Date Created
The date the item was original created (prior to any relationship with the ASU Digital Repositories.)
2024
Agent
- Author (aut): iyer, kaushik
- Thesis advisor (ths): Tsakalis, Konstantinos
- Committee member: Arenz, Christian
- Committee member: Redkar, Sangram
- Publisher (pbl): Arizona State University