Context recognition methods using audio signals for human-machine interaction
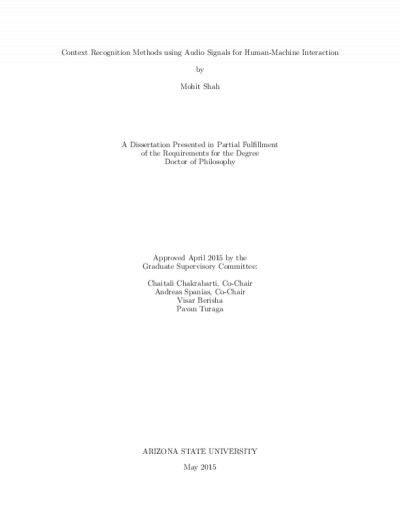
The expression and perception of emotions varies across speakers and cultures, thus, determining features and classification methods that generalize well to different conditions is strongly desired. A latent topic models-based method is proposed to learn supra-segmental features from low-level acoustic descriptors. The derived features outperform state-of-the-art approaches over multiple databases. Cross-corpus studies are conducted to determine the ability of these features to generalize well across different databases. The proposed method is also applied to derive features from facial expressions; a multi-modal fusion overcomes the deficiencies of a speech only approach and further improves the recognition performance.
Besides affecting the acoustic properties of speech, emotions have a strong influence over speech articulation kinematics. A learning approach, which constrains a classifier trained over acoustic descriptors, to also model articulatory data is proposed here. This method requires articulatory information only during the training stage, thus overcoming the challenges inherent to large-scale data collection, while simultaneously exploiting the correlations between articulation kinematics and acoustic descriptors to improve the accuracy of emotion recognition systems.
Identifying context from ambient sounds in a lifelogging scenario requires feature extraction, segmentation and annotation techniques capable of efficiently handling long duration audio recordings; a complete framework for such applications is presented. The performance is evaluated on real world data and accompanied by a prototypical Android-based user interface.
The proposed methods are also assessed in terms of computation and implementation complexity. Software and field programmable gate array based implementations are considered for emotion recognition, while virtual platforms are used to model the complexities of lifelogging. The derived metrics are used to determine the feasibility of these methods for applications requiring real-time capabilities and low power consumption.
- Author (aut): Shah, Mohit
- Thesis advisor (ths): Spanias, Andreas
- Thesis advisor (ths): Chakrabarti, Chaitali
- Committee member: Berisha, Visar
- Committee member: Turaga, Pavan
- Publisher (pbl): Arizona State University