Supporting Global Environmental Change Research: A Review of Trends and Knowledge Gaps in Urban Remote Sensing
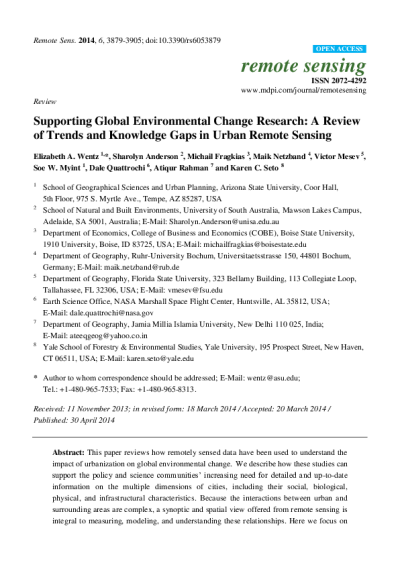
This paper reviews how remotely sensed data have been used to understand the impact of urbanization on global environmental change. We describe how these studies can support the policy and science communities’ increasing need for detailed and up-to-date information on the multiple dimensions of cities, including their social, biological, physical, and infrastructural characteristics. Because the interactions between urban and surrounding areas are complex, a synoptic and spatial view offered from remote sensing is integral to measuring, modeling, and understanding these relationships. Here we focus on three themes in urban remote sensing science: mapping, indices, and modeling. For mapping we describe the data sources, methods, and limitations of mapping urban boundaries, land use and land cover, population, temperature, and air quality. Second, we described how spectral information is manipulated to create comparative biophysical, social, and spatial indices of the urban environment. Finally, we focus how the mapped information and indices are used as inputs or parameters in models that measure changes in climate, hydrology, land use, and economics.
- Author (aut): Wentz, Elizabeth
- Author (aut): Anderson, Sharolyn
- Author (aut): Fragkias, Michail
- Author (aut): Netzband, Maik
- Author (aut): Mesev, Victor
- Author (aut): Myint, Soe
- Author (aut): Quattrochi, Dale
- Author (aut): Rahman, Atiqur
- Author (aut): Seto, Karen C.
- Contributor (ctb): College of Liberal Arts and Sciences