Multi-Camera Bird-Eye-View Occupancy Detection for Intelligent Transportation System
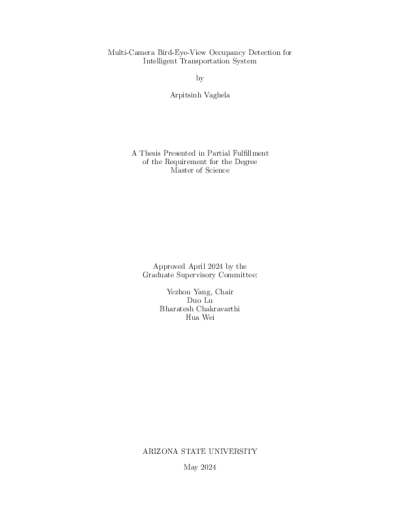
Description
3D perception poses a significant challenge in Intelligent Transportation Systems (ITS) due to occlusion and limited field of view. The necessity for real-time processing and alignment with existing traffic infrastructure compounds these limitations. To counter these issues, this work introduces a novel multi-camera Bird-Eye View (BEV) occupancy detection framework. This approach leverages multi-camera setups to overcome occlusion and field-of-view limitations while employing BEV occupancy to simplify the 3D perception task, ensuring critical information is retained. A noble dataset for BEV Occupancy detection, encompassing diverse scenes and varying camera configurations, was created using the CARLA simulator. Subsequent extensive evaluation of various Multiview occupancy detection models showcased the critical roles of scene diversity and occupancy grid resolution in enhancing model performance. A structured framework that complements the generated data is proposed for data collection in the real world. The trained model is validated against real-world conditions to ensure its practical application, demonstrating the influence of robust dataset design in refining ITS perception systems. This contributes to significant advancements in traffic management, safety, and operational efficiency.
Date Created
The date the item was original created (prior to any relationship with the ASU Digital Repositories.)
2024
Agent
- Author (aut): Vaghela, Arpitsinh Rohitkumar
- Thesis advisor (ths): Yang, Yezhou
- Committee member: Lu, Duo
- Committee member: Chakravarthi, Bharatesh
- Committee member: Wei, Hua
- Publisher (pbl): Arizona State University