Towards Addressing GAN Training Instabilities: Dual-Objective GANs with Tunable Parameters
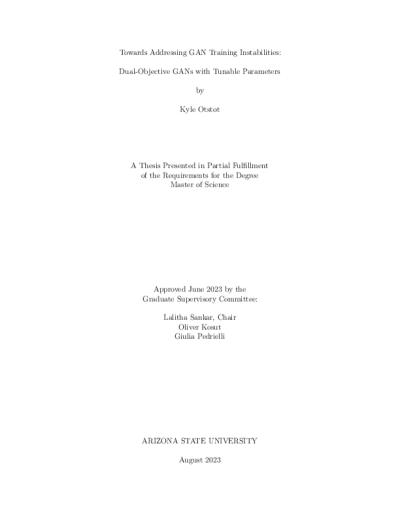
Description
Generative Adversarial Networks (GANs) have emerged as a powerful framework for generating realistic and high-quality data. In the original ``vanilla'' GAN formulation, two models -- the generator and discriminator -- are engaged in a min-max game and optimize the same value function. Despite offering an intuitive approach, vanilla GANs often face stability challenges such as vanishing gradients and mode collapse. Addressing these common failures, recent work has proposed the use of tunable classification losses in place of traditional value functions. Although parameterized robust loss families, e.g. $\alpha$-loss, have shown promising characteristics as value functions, this thesis argues that the generator and discriminator require separate objective functions to achieve their different goals. As a result, this thesis introduces the $(\alpha_{D}, \alpha_{G})$-GAN, a parameterized class of dual-objective GANs, as an alternative approach to the standard vanilla GAN. The $(\alpha_{D}, \alpha_{G})$-GAN formulation, inspired by $\alpha$-loss, allows practitioners to tune the parameters $(\alpha_{D}, \alpha_{G}) \in [0,\infty)^{2}$ to provide a more stable training process. The objectives for the generator and discriminator in $(\alpha_{D}, \alpha_{G})$-GAN are derived, and the advantages of using these objectives are investigated. In particular, the optimization trajectory of the generator is found to be influenced by the choice of $\alpha_{D}$ and $\alpha_{G}$. Empirical evidence is presented through experiments conducted on various datasets, including the 2D Gaussian Mixture Ring, Celeb-A image dataset, and LSUN Classroom image dataset. Performance metrics such as mode coverage and Fréchet Inception Distance (FID) are used to evaluate the effectiveness of the $(\alpha_{D}, \alpha_{G})$-GAN compared to the vanilla GAN and state-of-the-art Least Squares GAN (LSGAN). The experimental results demonstrate that tuning $\alpha_{D} < 1$ leads to improved stability, robustness to hyperparameter choice, and competitive performance compared to LSGAN.
Date Created
The date the item was original created (prior to any relationship with the ASU Digital Repositories.)
2023
Agent
- Author (aut): Otstot, Kyle
- Thesis advisor (ths): Sankar, Lalitha
- Committee member: Kosut, Oliver
- Committee member: Pedrielli, Giulia
- Publisher (pbl): Arizona State University