Brain-Based Authentication Systems and Brain Liveness Problem
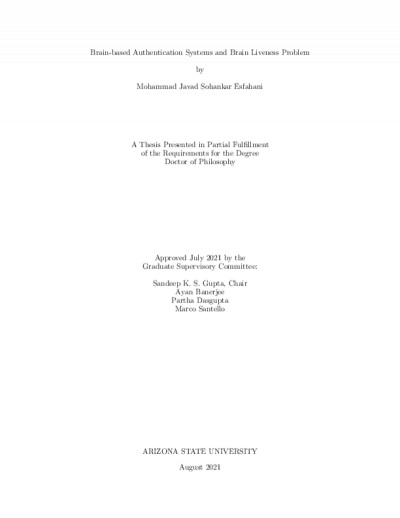
Description
In recent years, brain signals have gained attention as a potential trait for biometric-based security systems and laboratory systems have been designed. A real-world brain-based security system requires to be usable, accurate, and robust. While there have been developments in these aspects, there are still challenges to be met. With regard to usability, users need to provide lengthy amount of data compared to other traits such as fingerprint and face to get authenticated. Furthermore, in the majority of works, medical sensors are used which are more accurate compared to commercial ones but have a tedious setup process and are not mobile. Performance wise, the current state-of-art can provide acceptable accuracy on a small pool of users data collected in few sessions close to each other but still falls behind on a large pool of subjects over a longer time period. Finally, a brain security system should be robust against presentation attacks to prevent adversaries from gaining access to the system.
This dissertation proposes E-BIAS (EEG-based Identification and Authentication System), a brain-mobile security system that makes contributions in three directions. First, it provides high performance on signals with shorter lengths collected by commercial sensors and processed with lightweight models to meet the computation/energy capacity of mobile devices. Second, to evaluate the system's robustness a novel presentation attack was designed which challenged the literature's presumption of intrinsic liveness property for brain signals. Third, to bridge the gap, I formulated and studied the brain liveness problem and proposed two solution approaches (model-aware & model agnostic) to ensure liveness and enhance robustness against presentation attacks. Under each of the two solution approaches, several methods were suggested and evaluated against both synthetic and manipulative classes of attacks (a total of 43 different attack vectors). Methods in both model-aware and model-agnostic approaches were successful in achieving an error rate of zero (0%). More importantly, such error rates were reached in face of unseen attacks which provides evidence of the generalization potentials of the proposed solution approaches and methods. I suggested an adversarial workflow to facilitate attack and defense cycles to allow for enhanced generalization capacity for domains in which the decision-making process is non-deterministic such as cyber-physical systems (e.g. biometric/medical monitoring, autonomous machines, etc.). I utilized this workflow for the brain liveness problem and was able to iteratively improve the performance of both the designed attacks and the proposed liveness detection methods.
Date Created
The date the item was original created (prior to any relationship with the ASU Digital Repositories.)
2021
Agent
- Author (aut): Sohankar Esfahani, Mohammad Javad
- Thesis advisor (ths): Gupta, Sandeep K.S.
- Committee member: Santello, Marco
- Committee member: Dasgupta, Partha
- Committee member: Banerjee, Ayan
- Publisher (pbl): Arizona State University