BraiNet: A Framework for Designing Pervasive Brain-Machine Interface Applications
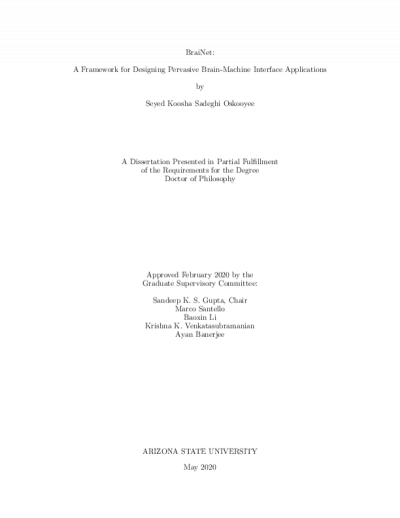
Description
Due to the advent of easy-to-use, portable, and cost-effective brain signal sensing devices, pervasive Brain-Machine Interface (BMI) applications using Electroencephalogram (EEG) are growing rapidly. The main objectives of these applications are: 1) pervasive collection of brain data from multiple users, 2) processing the collected data to recognize the corresponding mental states, and 3) providing real-time feedback to the end users, activating an actuator, or information harvesting by enterprises for further services. Developing BMI applications faces several challenges, such as cumbersome setup procedure, low signal-to-noise ratio, insufficient signal samples for analysis, and long processing times. Internet-of-Things (IoT) technologies provide the opportunity to solve these challenges through large scale data collection, fast data transmission, and computational offloading.
This research proposes an IoT-based framework, called BraiNet, that provides a standard design methodology for fulfilling the pervasive BMI applications requirements including: accuracy, timeliness, energy-efficiency, security, and dependability. BraiNet applies Machine Learning (ML) based solutions (e.g. classifiers and predictive models) to: 1) improve the accuracy of mental state detection on-the-go, 2) provide real-time feedback to the users, and 3) save power on mobile platforms. However, BraiNet inherits security breaches of IoT, due to applying off-the-shelf soft/hardware, high accessibility, and massive network size. ML algorithms, as the core technology for mental state recognition, are among the main targets for cyber attackers. Novel ML security solutions are proposed and added to BraiNet, which provide analytical methodologies for tuning the ML hyper-parameters to be secure against attacks.
To implement these solutions, two main optimization problems are solved: 1) maximizing accuracy, while minimizing delays and power consumption, and 2) maximizing the ML security, while keeping its accuracy high. Deep learning algorithms, delay and power models are developed to solve the former problem, while gradient-free optimization techniques, such as Bayesian optimization are applied for the latter. To test the framework, several BMI applications are implemented, such as EEG-based drivers fatigue detector (SafeDrive), EEG-based identification and authentication system (E-BIAS), and interactive movies that adapt to viewers mental states (nMovie). The results from the experiments on the implemented applications show the successful design of pervasive BMI applications based on the BraiNet framework.
This research proposes an IoT-based framework, called BraiNet, that provides a standard design methodology for fulfilling the pervasive BMI applications requirements including: accuracy, timeliness, energy-efficiency, security, and dependability. BraiNet applies Machine Learning (ML) based solutions (e.g. classifiers and predictive models) to: 1) improve the accuracy of mental state detection on-the-go, 2) provide real-time feedback to the users, and 3) save power on mobile platforms. However, BraiNet inherits security breaches of IoT, due to applying off-the-shelf soft/hardware, high accessibility, and massive network size. ML algorithms, as the core technology for mental state recognition, are among the main targets for cyber attackers. Novel ML security solutions are proposed and added to BraiNet, which provide analytical methodologies for tuning the ML hyper-parameters to be secure against attacks.
To implement these solutions, two main optimization problems are solved: 1) maximizing accuracy, while minimizing delays and power consumption, and 2) maximizing the ML security, while keeping its accuracy high. Deep learning algorithms, delay and power models are developed to solve the former problem, while gradient-free optimization techniques, such as Bayesian optimization are applied for the latter. To test the framework, several BMI applications are implemented, such as EEG-based drivers fatigue detector (SafeDrive), EEG-based identification and authentication system (E-BIAS), and interactive movies that adapt to viewers mental states (nMovie). The results from the experiments on the implemented applications show the successful design of pervasive BMI applications based on the BraiNet framework.
Date Created
The date the item was original created (prior to any relationship with the ASU Digital Repositories.)
2020
Agent
- Author (aut): Sadeghi Oskooyee, Seyed Koosha
- Thesis advisor (ths): Gupta, Sandeep K S
- Committee member: Santello, Marco
- Committee member: Li, Baoxin
- Committee member: Venkatasubramanian, Krishna K
- Committee member: Banerjee, Ayan
- Publisher (pbl): Arizona State University