Learning from task heterogeneity in social media
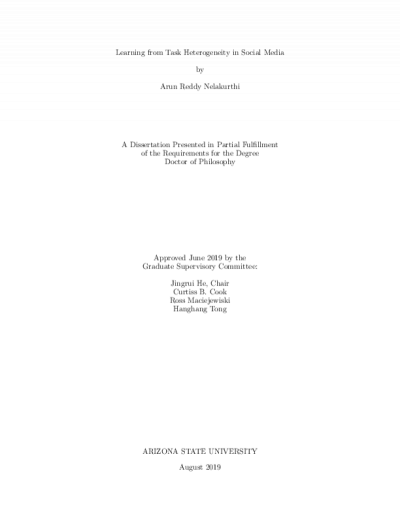
Description
In recent years, the rise in social media usage both vertically in terms of the number of users by platform and horizontally in terms of the number of platforms per user has led to data explosion.
User-generated social media content provides an excellent opportunity to mine data of interest and to build resourceful applications. The rise in the number of healthcare-related social media platforms and the volume of healthcare knowledge available online in the last decade has resulted in increased social media usage for personal healthcare. In the United States, nearly ninety percent of adults, in the age group 50-75, have used social media to seek and share health information. Motivated by the growth of social media usage, this thesis focuses on healthcare-related applications, study various challenges posed by social media data, and address them through novel and effective machine learning algorithms.
The major challenges for effectively and efficiently mining social media data to build functional applications include: (1) Data reliability and acceptance: most social media data (especially in the context of healthcare-related social media) is not regulated and little has been studied on the benefits of healthcare-specific social media; (2) Data heterogeneity: social media data is generated by users with both demographic and geographic diversity; (3) Model transparency and trustworthiness: most existing machine learning models for addressing heterogeneity are considered as black box models, not many providing explanations for why they do what they do to trust them.
In response to these challenges, three main research directions have been investigated in this thesis: (1) Analyzing social media influence on healthcare: to study the real world impact of social media as a source to offer or seek support for patients with chronic health conditions; (2) Learning from task heterogeneity: to propose various models and algorithms that are adaptable to new social media platforms and robust to dynamic social media data, specifically on modeling user behaviors, identifying similar actors across platforms, and adapting black box models to a specific learning scenario; (3) Explaining heterogeneous models: to interpret predictive models in the presence of task heterogeneity. In this thesis, novel algorithms with theoretical analysis from various aspects (e.g., time complexity, convergence properties) have been proposed. The effectiveness and efficiency of the proposed algorithms is demonstrated by comparison with state-of-the-art methods and relevant case studies.
User-generated social media content provides an excellent opportunity to mine data of interest and to build resourceful applications. The rise in the number of healthcare-related social media platforms and the volume of healthcare knowledge available online in the last decade has resulted in increased social media usage for personal healthcare. In the United States, nearly ninety percent of adults, in the age group 50-75, have used social media to seek and share health information. Motivated by the growth of social media usage, this thesis focuses on healthcare-related applications, study various challenges posed by social media data, and address them through novel and effective machine learning algorithms.
The major challenges for effectively and efficiently mining social media data to build functional applications include: (1) Data reliability and acceptance: most social media data (especially in the context of healthcare-related social media) is not regulated and little has been studied on the benefits of healthcare-specific social media; (2) Data heterogeneity: social media data is generated by users with both demographic and geographic diversity; (3) Model transparency and trustworthiness: most existing machine learning models for addressing heterogeneity are considered as black box models, not many providing explanations for why they do what they do to trust them.
In response to these challenges, three main research directions have been investigated in this thesis: (1) Analyzing social media influence on healthcare: to study the real world impact of social media as a source to offer or seek support for patients with chronic health conditions; (2) Learning from task heterogeneity: to propose various models and algorithms that are adaptable to new social media platforms and robust to dynamic social media data, specifically on modeling user behaviors, identifying similar actors across platforms, and adapting black box models to a specific learning scenario; (3) Explaining heterogeneous models: to interpret predictive models in the presence of task heterogeneity. In this thesis, novel algorithms with theoretical analysis from various aspects (e.g., time complexity, convergence properties) have been proposed. The effectiveness and efficiency of the proposed algorithms is demonstrated by comparison with state-of-the-art methods and relevant case studies.
Date Created
The date the item was original created (prior to any relationship with the ASU Digital Repositories.)
2019
Agent
- Author (aut): Nelakurthi, Arun Reddy
- Thesis advisor (ths): He, Jingrui
- Committee member: Cook, Curtiss B
- Committee member: Maciejewski, Ross
- Committee member: Tong, Hanghang
- Publisher (pbl): Arizona State University