Bayesian Inference for Markov Kernels Valued in Wasserstein Spaces
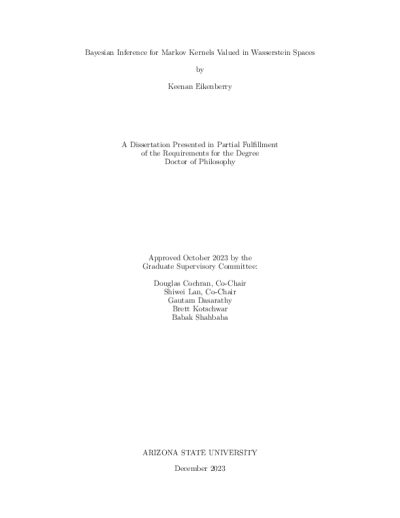
Description
In this work, the author analyzes quantitative and structural aspects of Bayesian inference using Markov kernels, Wasserstein metrics, and Kantorovich monads. In particular, the author shows the following main results: first, that Markov kernels can be viewed as Borel measurable maps with values in a Wasserstein space; second, that the Disintegration Theorem can be interpreted as a literal equality of integrals using an original theory of integration for Markov kernels; third, that the Kantorovich monad can be defined for Wasserstein metrics of any order; and finally, that, under certain assumptions, a generalized Bayes’s Law for Markov kernels provably leads to convergence of the expected posterior distribution in the Wasserstein metric. These contributions provide a basis for studying further convergence, approximation, and stability properties of Bayesian inverse maps and inference processes using a unified theoretical framework that bridges between statistical inference, machine learning, and probabilistic programming semantics.
Date Created
The date the item was original created (prior to any relationship with the ASU Digital Repositories.)
2023
Agent
- Author (aut): Eikenberry, Keenan
- Thesis advisor (ths): Cochran, Douglas
- Thesis advisor (ths): Lan, Shiwei
- Committee member: Dasarathy, Gautam
- Committee member: Kotschwar, Brett
- Committee member: Shahbaba, Babak
- Publisher (pbl): Arizona State University