Feature selection techniques for effective model building and estimation on Twitter data to understand the political scenario in Latvia with supporting visualizations
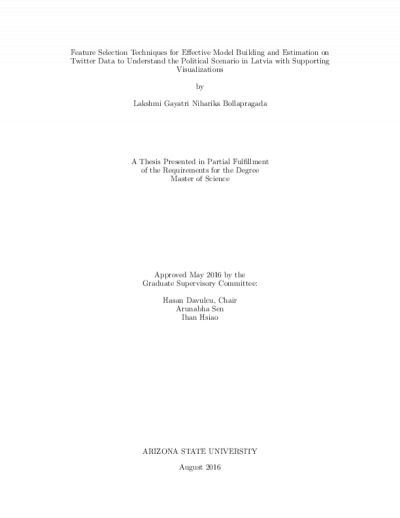
Description
In supervised learning, machine learning techniques can be applied to learn a model on
a small set of labeled documents which can be used to classify a larger set of unknown
documents. Machine learning techniques can be used to analyze a political scenario
in a given society. A lot of research has been going on in this field to understand
the interactions of various people in the society in response to actions taken by their
organizations.
This paper talks about understanding the Russian influence on people in Latvia.
This is done by building an eeffective model learnt on initial set of documents
containing a combination of official party web-pages, important political leaders' social
networking sites. Since twitter is a micro-blogging site which allows people to post
their opinions on any topic, the model built is used for estimating the tweets sup-
porting the Russian and Latvian political organizations in Latvia. All the documents
collected for analysis are in Latvian and Russian languages which are rich in vocabulary resulting into huge number of features. Hence, feature selection techniques can
be used to reduce the vocabulary set relevant to the classification model. This thesis
provides a comparative analysis of traditional feature selection techniques and implementation of a new iterative feature selection method using EM and cross-domain
training along with supportive visualization tool. This method out performed other
feature selection methods by reducing the number of features up-to 50% along with
good model accuracy. The results from the classification are used to interpret user
behavior and their political influence patterns across organizations in Latvia using
interactive dashboard with combination of powerful widgets.
a small set of labeled documents which can be used to classify a larger set of unknown
documents. Machine learning techniques can be used to analyze a political scenario
in a given society. A lot of research has been going on in this field to understand
the interactions of various people in the society in response to actions taken by their
organizations.
This paper talks about understanding the Russian influence on people in Latvia.
This is done by building an eeffective model learnt on initial set of documents
containing a combination of official party web-pages, important political leaders' social
networking sites. Since twitter is a micro-blogging site which allows people to post
their opinions on any topic, the model built is used for estimating the tweets sup-
porting the Russian and Latvian political organizations in Latvia. All the documents
collected for analysis are in Latvian and Russian languages which are rich in vocabulary resulting into huge number of features. Hence, feature selection techniques can
be used to reduce the vocabulary set relevant to the classification model. This thesis
provides a comparative analysis of traditional feature selection techniques and implementation of a new iterative feature selection method using EM and cross-domain
training along with supportive visualization tool. This method out performed other
feature selection methods by reducing the number of features up-to 50% along with
good model accuracy. The results from the classification are used to interpret user
behavior and their political influence patterns across organizations in Latvia using
interactive dashboard with combination of powerful widgets.
Date Created
The date the item was original created (prior to any relationship with the ASU Digital Repositories.)
2016
Agent
- Author (aut): Bollapragada, Lakshmi Gayatri Niharika
- Thesis advisor (ths): Davulcu, Hasan
- Committee member: Sen, Arunabha
- Committee member: Hsiao, Ihan
- Publisher (pbl): Arizona State University