A Statistical Framework for Detecting Edges from Noisy Fourier Data with Multiple Concentration Factors
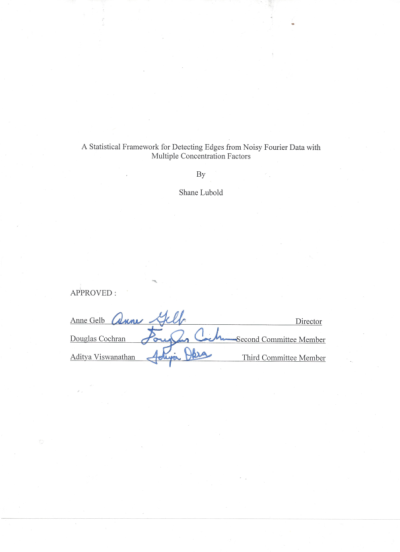
Description
The concentration factor edge detection method was developed to compute the locations and values of jump discontinuities in a piecewise-analytic function from its first few Fourier series coecients. The method approximates the singular support of a piecewise smooth function using an altered Fourier conjugate partial sum. The accuracy and characteristic features of the resulting jump function approximation depends on these lters, known as concentration factors. Recent research showed that that these concentration factors could be designed using aexible iterative framework, improving upon the overall accuracy and robustness of the method, especially in the case where some Fourier data are untrustworthy or altogether missing. Hypothesis testing methods were used to determine how well the original concentration factor method could locate edges using noisy Fourier data. This thesis combines the iterative design aspect of concentration factor design and hypothesis testing by presenting a new algorithm that incorporates multiple concentration factors into one statistical test, which proves more ective at determining jump discontinuities than the previous HT methods. This thesis also examines how the quantity and location of Fourier data act the accuracy of HT methods. Numerical examples are provided.
Date Created
The date the item was original created (prior to any relationship with the ASU Digital Repositories.)
2016-05
Agent
- Author (aut): Lubold, Shane Michael
- Thesis director: Gelb, Anne
- Committee member: Cochran, Doug
- Committee member: Viswanathan, Aditya
- Contributor (ctb): Economics Program in CLAS
- Contributor (ctb): School of Mathematical and Statistical Sciences
- Contributor (ctb): Barrett, The Honors College