Data Poisoning Attacks on Linked Data with Graph Regularization
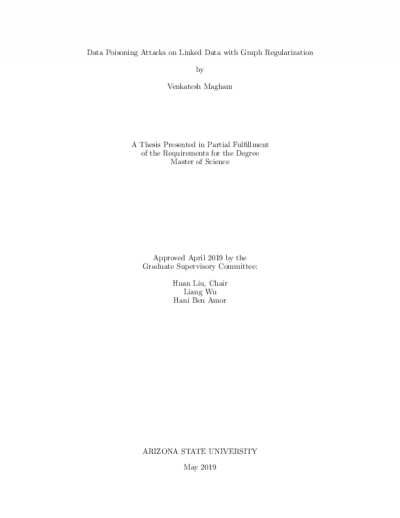
Description
Social media has become the norm of everyone for communication. The usage of social media has increased exponentially in the last decade. The myriads of Social media services such as Facebook, Twitter, Snapchat, and Instagram etc allow people to connect with their friends, and followers freely. The attackers who try to take advantage of this situation has also increased at an exponential rate. Every social media service has its own recommender systems and user profiling algorithms. These algorithms use users current information to make different recommendations. Often the data that is formed from social media services is Linked data as each item/user is usually linked with other users/items. Recommender systems due to their ubiquitous and prominent nature are prone to several forms of attacks. One of the major form of attacks is poisoning the training set data. As recommender systems use current user/item information as the training set to make recommendations, the attacker tries to modify the training set in such a way that the recommender system would benefit the attacker or give incorrect recommendations and hence failing in its basic functionality. Most existing training set attack algorithms work with ``flat" attribute-value data which is typically assumed to be independent and identically distributed (i.i.d.). However, the i.i.d. assumption does not hold for social media data since it is inherently linked as described above. Usage of user-similarity with Graph Regularizer in morphing the training data produces best results to attacker. This thesis proves the same by demonstrating with experiments on Collaborative Filtering with multiple datasets.
Date Created
The date the item was original created (prior to any relationship with the ASU Digital Repositories.)
2019
Agent
- Author (aut): Magham, Venkatesh
- Thesis advisor (ths): Liu, Huan
- Committee member: Wu, Liang
- Committee member: Amor, Hani Ben
- Publisher (pbl): Arizona State University