Generating Vocabulary Sets for Implicit Language Learning using Masked Language Modeling
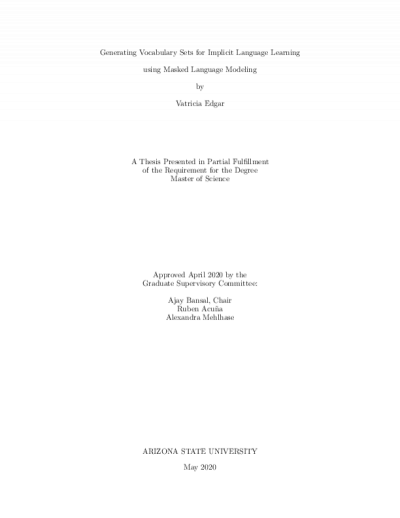
Description
Globalization is driving a rapid increase in motivation for learning new languages, with online and mobile language learning applications being an extremely popular method of doing so. Many language learning applications focus almost exclusively on aiding students in acquiring vocabulary, one of the most important elements in achieving fluency in a language. A well-balanced language curriculum must include both explicit vocabulary instruction and implicit vocabulary learning through interaction with authentic language materials. However, most language learning applications focus only on explicit instruction, providing little support for implicit learning. Students require support with implicit vocabulary learning because they need enough context to guess and acquire new words. Traditional techniques aim to teach students enough vocabulary to comprehend the text, thus enabling them to acquire new words. Despite the wide variety of support for vocabulary learning offered by learning applications today, few offer guidance on how to select an optimal vocabulary study set.
This thesis proposes a novel method of student modeling which uses pre-trained masked language models to model a student's reading comprehension abilities and detect words which are required for comprehension of a text. It explores the efficacy of using pre-trained masked language models to model human reading comprehension and presents a vocabulary study set generation pipeline using this method. This pipeline creates vocabulary study sets for explicit language learning that enable comprehension while still leaving some words to be acquired implicitly. Promising results show that masked language modeling can be used to model human comprehension and that the pipeline produces reasonably sized vocabulary study sets.
This thesis proposes a novel method of student modeling which uses pre-trained masked language models to model a student's reading comprehension abilities and detect words which are required for comprehension of a text. It explores the efficacy of using pre-trained masked language models to model human reading comprehension and presents a vocabulary study set generation pipeline using this method. This pipeline creates vocabulary study sets for explicit language learning that enable comprehension while still leaving some words to be acquired implicitly. Promising results show that masked language modeling can be used to model human comprehension and that the pipeline produces reasonably sized vocabulary study sets.
Date Created
The date the item was original created (prior to any relationship with the ASU Digital Repositories.)
2020
Agent
- Author (aut): Edgar, Vatricia Cathrine
- Thesis advisor (ths): Bansal, Ajay
- Committee member: Acuna, Ruben
- Committee member: Mehlhase, Alexandra
- Publisher (pbl): Arizona State University