Diffusion in Networks: Source Localization, History Reconstruction and Real-Time Network Robustification
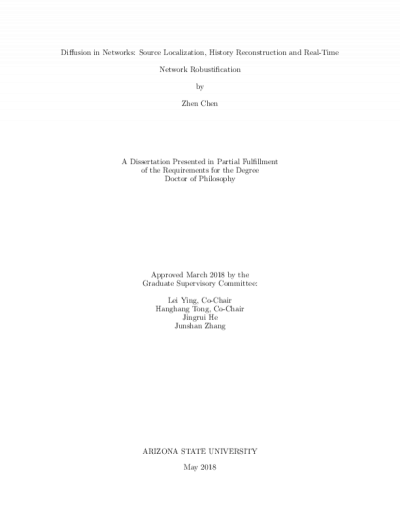
Description
Diffusion processes in networks can be used to model many real-world processes, such as the propagation of a rumor on social networks and cascading failures on power networks. Analysis of diffusion processes in networks can help us answer important questions such as the role and the importance of each node in the network for spreading the diffusion and how to top or contain a cascading failure in the network. This dissertation consists of three parts.
In the first part, we study the problem of locating multiple diffusion sources in networks under the Susceptible-Infected-Recovered (SIR) model. Given a complete snapshot of the network, we developed a sample-path-based algorithm, named clustering and localization, and proved that for regular trees, the estimators produced by the proposed algorithm are within a constant distance from the real sources with a high probability. Then, we considered the case in which only a partial snapshot is observed and proposed a new algorithm, named Optimal-Jordan-Cover (OJC). The algorithm first extracts a subgraph using a candidate selection algorithm that selects source candidates based on the number of observed infected nodes in their neighborhoods. Then, in the extracted subgraph, OJC finds a set of nodes that "cover" all observed infected nodes with the minimum radius. The set of nodes is called the Jordan cover, and is regarded as the set of diffusion sources. We proved that OJC can locate all sources with probability one asymptotically with partial observations in the Erdos-Renyi (ER) random graph. Multiple experiments on different networks were done, which show our algorithms outperform others.
In the second part, we tackle the problem of reconstructing the diffusion history from partial observations. We formulated the diffusion history reconstruction problem as a maximum a posteriori (MAP) problem and proved the problem is NP hard. Then we proposed a step-by- step reconstruction algorithm, which can always produce a diffusion history that is consistent with the partial observations. Our experimental results based on synthetic and real networks show that the algorithm significantly outperforms some existing methods.
In the third part, we consider the problem of improving the robustness of an interdependent network by rewiring a small number of links during a cascading attack. We formulated the problem as a Markov decision process (MDP) problem. While the problem is NP-hard, we developed an effective and efficient algorithm, RealWire, to robustify the network and to mitigate the damage during the attack. Extensive experimental results show that our algorithm outperforms other algorithms on most of the robustness metrics.
In the first part, we study the problem of locating multiple diffusion sources in networks under the Susceptible-Infected-Recovered (SIR) model. Given a complete snapshot of the network, we developed a sample-path-based algorithm, named clustering and localization, and proved that for regular trees, the estimators produced by the proposed algorithm are within a constant distance from the real sources with a high probability. Then, we considered the case in which only a partial snapshot is observed and proposed a new algorithm, named Optimal-Jordan-Cover (OJC). The algorithm first extracts a subgraph using a candidate selection algorithm that selects source candidates based on the number of observed infected nodes in their neighborhoods. Then, in the extracted subgraph, OJC finds a set of nodes that "cover" all observed infected nodes with the minimum radius. The set of nodes is called the Jordan cover, and is regarded as the set of diffusion sources. We proved that OJC can locate all sources with probability one asymptotically with partial observations in the Erdos-Renyi (ER) random graph. Multiple experiments on different networks were done, which show our algorithms outperform others.
In the second part, we tackle the problem of reconstructing the diffusion history from partial observations. We formulated the diffusion history reconstruction problem as a maximum a posteriori (MAP) problem and proved the problem is NP hard. Then we proposed a step-by- step reconstruction algorithm, which can always produce a diffusion history that is consistent with the partial observations. Our experimental results based on synthetic and real networks show that the algorithm significantly outperforms some existing methods.
In the third part, we consider the problem of improving the robustness of an interdependent network by rewiring a small number of links during a cascading attack. We formulated the problem as a Markov decision process (MDP) problem. While the problem is NP-hard, we developed an effective and efficient algorithm, RealWire, to robustify the network and to mitigate the damage during the attack. Extensive experimental results show that our algorithm outperforms other algorithms on most of the robustness metrics.
Date Created
The date the item was original created (prior to any relationship with the ASU Digital Repositories.)
2018
Agent
- Author (aut): Chen, Zhen
- Thesis advisor (ths): Ying, Lei
- Thesis advisor (ths): Tong, Hanghang
- Committee member: Zhang, Junshan
- Committee member: He, Jingrui
- Publisher (pbl): Arizona State University