A Novel Battery Management & Charging Solution for Autonomous UAV Systems
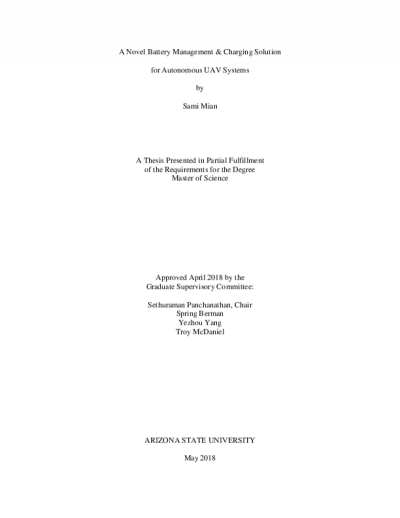
Description
Currently, one of the biggest limiting factors for long-term deployment of autonomous systems is the power constraints of a platform. In particular, for aerial robots such as unmanned aerial vehicles (UAVs), the energy resource is the main driver of mission planning and operation definitions, as everything revolved around flight time. The focus of this work is to develop a new method of energy storage and charging for autonomous UAV systems, for use during long-term deployments in a constrained environment. We developed a charging solution that allows pre-equipped UAV system to land on top of designated charging pads and rapidly replenish their battery reserves, using a contact charging point. This system is designed to work with all types of rechargeable batteries, focusing on Lithium Polymer (LiPo) packs, that incorporate a battery management system for increased reliability. The project also explores optimization methods for fleets of UAV systems, to increase charging efficiency and extend battery lifespans. Each component of this project was first designed and tested in computer simulation. Following positive feedback and results, prototypes for each part of this system were developed and rigorously tested. Results show that the contact charging method is able to charge LiPo batteries at a 1-C rate, which is the industry standard rate, maintaining the same safety and efficiency standards as modern day direct connection chargers. Control software for these base stations was also created, to be integrated with a fleet management system, and optimizes UAV charge levels and distribution to extend LiPo battery lifetimes while still meeting expected mission demand. Each component of this project (hardware/software) was designed for manufacturing and implementation using industry standard tools, making it ideal for large-scale implementations. This system has been successfully tested with a fleet of UAV systems at Arizona State University, and is currently being integrated into an Arizona smart city environment for deployment.
Date Created
The date the item was original created (prior to any relationship with the ASU Digital Repositories.)
2018
Agent
- Author (aut): Mian, Sami
- Thesis advisor (ths): Panchanathan, Sethuraman
- Committee member: Berman, Spring
- Committee member: Yang, Yezhou
- Committee member: McDaniel, Troy
- Publisher (pbl): Arizona State University