Convolutional Neural Network for Pose Initialization with Uncertainty Estimation
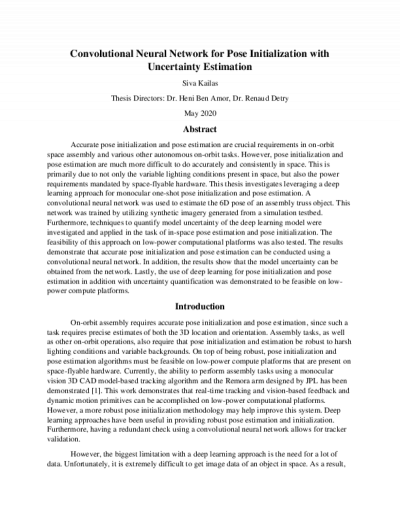
Description
Accurate pose initialization and pose estimation are crucial requirements in on-orbit space assembly and various other autonomous on-orbit tasks. However, pose initialization and pose estimation are much more difficult to do accurately and consistently in space. This is primarily due to not only the variable lighting conditions present in space, but also the power requirements mandated by space-flyable hardware. This thesis investigates leveraging a deep learning approach for monocular one-shot pose initialization and pose estimation. A convolutional neural network was used to estimate the 6D pose of an assembly truss object. This network was trained by utilizing synthetic imagery generated from a simulation testbed. Furthermore, techniques to quantify model uncertainty of the deep learning model were investigated and applied in the task of in-space pose estimation and pose initialization. The feasibility of this approach on low-power computational platforms was also tested. The results demonstrate that accurate pose initialization and pose estimation can be conducted using a convolutional neural network. In addition, the results show that the model uncertainty can be obtained from the network. Lastly, the use of deep learning for pose initialization and pose estimation in addition with uncertainty quantification was demonstrated to be feasible on low-power compute platforms.
Date Created
The date the item was original created (prior to any relationship with the ASU Digital Repositories.)
2020-05
Agent
- Author (aut): Kailas, Siva Maneparambil
- Thesis director: Ben Amor, Heni
- Committee member: Detry, Renaud
- Contributor (ctb): Economics Program in CLAS
- Contributor (ctb): School of Mathematical and Statistical Sciences
- Contributor (ctb): Computer Science and Engineering Program
- Contributor (ctb): Barrett, The Honors College