Graph Regularized Linear Regression
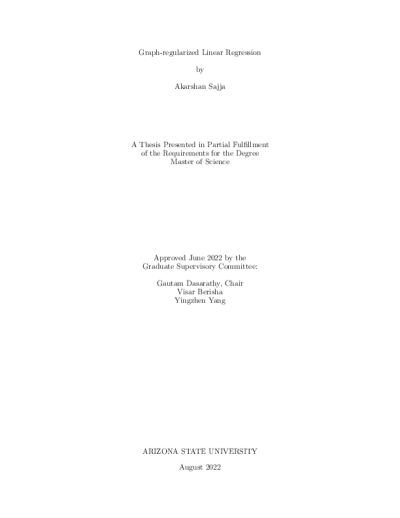
- Author (aut): Sajja, Akarshan
- Thesis advisor (ths): Dasarathy, Gautam
- Committee member: Berisha, Visar
- Committee member: Yang, Yingzhen
- Publisher (pbl): Arizona State University
In this research, I surveyed existing methods of characterizing Epilepsy from Electroencephalogram (EEG) data, including the Random Forest algorithm, which was claimed by many researchers to be the most effective at detecting epileptic seizures [7]. I observed that although many papers claimed a detection of >99% using Random Forest, it was not specified “when” the detection was declared within the 23.6 second interval of the seizure event. In this research, I created a time-series procedure to detect the seizure as early as possible within the 23.6 second epileptic seizure window and found that the detection is effective (> 92%) as early as the first few seconds of the epileptic episode. I intend to use this research as a stepping stone towards my upcoming Masters thesis research where I plan to expand the time-series detection mechanism to the pre-ictal stage, which will require a different dataset.
Spatial audio can be especially useful for directing human attention. However, delivering spatial audio through speakers, rather than headphones that deliver audio directly to the ears, produces the issue of crosstalk, where sounds from each of the two speakers reach the opposite ear, inhibiting the spatialized effect. A research team at Meteor Studio has developed an algorithm called Xblock that solves this issue using a crosstalk cancellation technique. This thesis project expands upon the existing Xblock IoT system by providing a way to test the accuracy of the directionality of sounds generated with spatial audio. More specifically, the objective is to determine whether the usage of Xblock with smart speakers can provide generalized audio localization, which refers to the ability to detect a general direction of where a sound might be coming from. This project also expands upon the existing Xblock technique to integrate voice commands, where users can verbalize the name of a lost item using the phrase, “Find [item]”, and the IoT system will use spatial audio to guide them to it.
Spatial audio can be especially useful for directing human attention. However, delivering spatial audio through speakers, rather than headphones that deliver audio directly to the ears, produces the issue of crosstalk, where sounds from each of the two speakers reach the opposite ear, inhibiting the spatialized effect. A research team at Meteor Studio has developed an algorithm called Xblock that solves this issue using a crosstalk cancellation technique. This thesis project expands upon the existing Xblock IoT system by providing a way to test the accuracy of the directionality of sounds generated with spatial audio. More specifically, the objective is to determine whether the usage of Xblock with smart speakers can provide generalized audio localization, which refers to the ability to detect a general direction of where a sound might be coming from. This project also expands upon the existing Xblock technique to integrate voice commands, where users can verbalize the name of a lost item using the phrase, “Find [item]”, and the IoT system will use spatial audio to guide them to it.