From SLAM to Spatial AI: Using Implicit 3D Latent Space Landmark Reconstruction for Robot Localization and Mapping
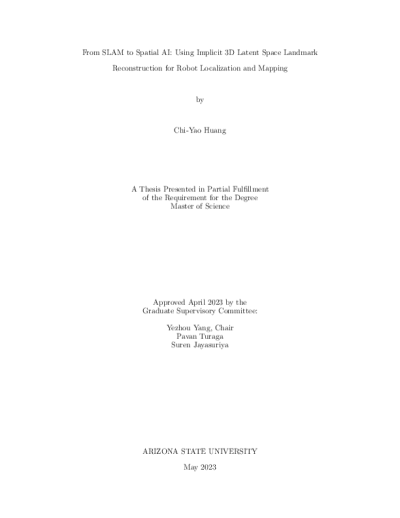
Description
Simultaneous localization and mapping (SLAM) has traditionally relied on low-level geometric or optical features. However, these features-based SLAM methods often struggle with feature-less or repetitive scenes. Additionally, low-level features may not provide sufficient information for robot navigation and manipulation, leaving robots without a complete understanding of the 3D spatial world. Advanced information is necessary to address these limitations. Fortunately, recent developments in learning-based 3D reconstruction allow robots to not only detect semantic meanings, but also recognize the 3D structure of objects from a few images. By combining this 3D structural information, SLAM can be improved from a low-level approach to a structure-aware approach. This work propose a novel approach for multi-view 3D reconstruction using recurrent transformer. This approach allows robots to accumulate information from multiple views and encode them into a compact latent space. The resulting latent representations are then decoded to produce 3D structural landmarks, which can be used to improve robot localization and mapping.
Date Created
The date the item was original created (prior to any relationship with the ASU Digital Repositories.)
2023
Agent
- Author (aut): Huang, Chi-Yao
- Thesis advisor (ths): Yang, Yezhou
- Committee member: Turaga, Pavan
- Committee member: Jayasuriya, Suren
- Publisher (pbl): Arizona State University