Neural Fields for Tomographic Imaging: with Applications in X-ray Computed Tomography and Synthetic Aperture Sonar
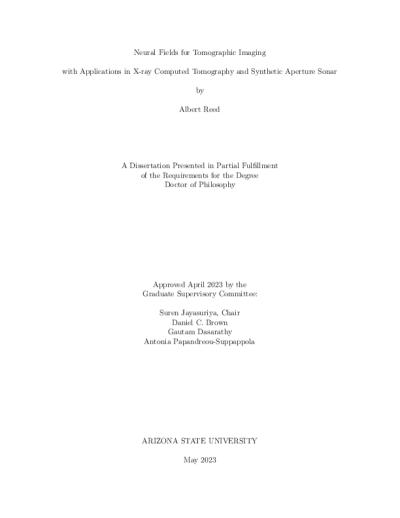
Description
Computed tomography (CT) and synthetic aperture sonar (SAS) are tomographic imaging techniques that are fundamental for applications within medical and remote sensing. Despite their successes, a number of factors constrain their image quality. For example, a time-varying scene during measurement acquisition yields image artifacts. Additionally, factors such as bandlimited or sparse measurements limit image resolution. This thesis presents novel algorithms and techniques to account for these factors during image formation and outperform traditional reconstruction methods. In particular, this thesis formulates analysis-by-synthesis optimizations that leverage neural fields to predict the scene and differentiable physics models that incorporate prior knowledge about image formation. The specific contributions include: (1) a method for reconstructing CT measurements from time-varying (non-stationary) scenes; (2) a method for deconvolving SAS images, which benefits image quality; (3) a method that couples neural fields and a differentiable acoustic model for 3D SAS reconstructions.
Date Created
The date the item was original created (prior to any relationship with the ASU Digital Repositories.)
2023
Agent
- Author (aut): Reed, Albert William
- Thesis advisor (ths): Jayasuriya, Suren
- Committee member: Brown, Daniel C
- Committee member: Dasarathy, Gautam
- Committee member: Papandreou-Suppappola, Antonia
- Publisher (pbl): Arizona State University