Discrete Approximations in Service Systems, Workforce Management, and Scheduling Models with Nonlinear Dynamics
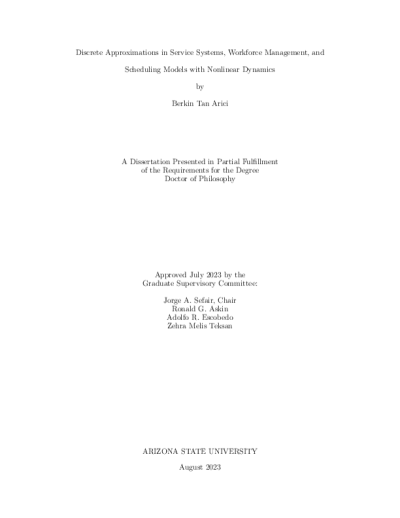
Description
Workforce planning in service systems is essential for customer satisfaction and profitability. Typical decisions include hiring levels, shift design, break scheduling, movement of workers around facilities, and matching worker skills to the requirements of tasks. The complexity of these decisions grows when realistic factors such as spatiotemporal demand dynamics, system performance assessment, or skill learning are incorporated into planning. As a result, optimal (or near-optimal) workforce plans should utilize resources efficiently and achieve satisfactory levels of service. This dissertation provides models and solution algorithms for three problems in service system optimization, which include realistic workforce management planning, nonlinear dynamics, and scheduling of tasks. The second chapter studies an airport security screening process and prescribes a daily operational plan, including service rate (i.e., number of servers), scheduling, and resource allocation decisions. The non-stationary arrivals are predicted and known in advance. A discrete-time queuing model that relies on a simple approximation and flow conservation equations embedded in a mixed-integer programming formulation, where consecutive time periods are connected. A multi-step solution method is proposed to optimize various metrics, such as maximum allowed queue lengths and wait times.
The third chapter extends the model from the second chapter. In this case, resources can move between different server locations, and their transit time is unproductive. Movement dynamics are captured via a multi-commodity flow model on a time-expanded network. A new discrete-time queue approximation scheme addresses the inaccuracies in existing methods stemming from server overload and underload fluctuations. Problem-specific valid inequalities are derived to improve the solution time, and a temporal decomposition algorithm is proposed to find initial feasible solutions.
The last chapter focuses on a medium to long-term scheduling problem with embedded workforce management decisions. The classic formulation for multi-mode multi-skill resource-constrained project scheduling problem is extended to incorporate worker task learning and training dynamics simultaneously. A discretization scheme to model the nonlinear learning process resulting from job assignments is developed. Training of workers is enabled to acquire new skills. A mixed-integer programming formulation is introduced, and a sequential solution scheme is proposed. The trade-offs between project duration and workforce skill composition objectives are investigated.
Date Created
The date the item was original created (prior to any relationship with the ASU Digital Repositories.)
2023
Agent
- Author (aut): Arici, Berkin Tan
- Thesis advisor (ths): Sefair, Jorge A
- Committee member: Askin, Ronald G
- Committee member: Escobedo, Adolfo R
- Committee member: Teksan, Zehra M
- Publisher (pbl): Arizona State University