Monocular Visual Odometry: Deep Learning vs Classical Approaches
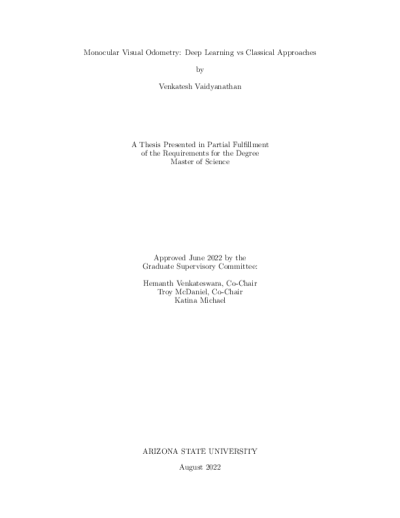
Description
Visual Odometry is one of the key aspects of robotic localization and mapping. Visual Odometry consists of many geometric-based approaches that convert visual data (images) into pose estimates of where the robot is in space. The classical geometric methods have shown promising results; they are carefully crafted and built explicitly for these tasks. However, such geometric methods require extreme fine-tuning and extensive prior knowledge to set up these systems for different scenarios. Classical Geometric approaches also require significant post-processing and optimization to minimize the error between the estimated pose and the global truth. In this body of work, the deep learning model was formed by combining SuperPoint and SuperGlue. The resulting model does not require any prior fine-tuning. It has been trained to enable both outdoor and indoor settings. The proposed deep learning model is applied to the Karlsruhe Institute of Technology and Toyota Technological Institute dataset along with other classical geometric visual odometry models. The proposed deep learning model has not been trained on the Karlsruhe Institute of Technology and Toyota Technological Institute dataset. It is only during experimentation that the deep learning model is first introduced to the Karlsruhe Institute of Technology and Toyota Technological Institute dataset. Using the monocular grayscale images from the visual odometer files of the Karlsruhe Institute of Technology and Toyota Technological Institute dataset, through the experiment to test the viability of the models for different sequences. The experiment has been performed on eight different sequences and has obtained the Absolute Trajectory Error and the time taken for each sequence to finish the computation. From the obtained results, there are inferences drawn from the classical and deep learning approaches.
Date Created
The date the item was original created (prior to any relationship with the ASU Digital Repositories.)
2022
Agent
- Author (aut): Vaidyanathan, Venkatesh
- Thesis advisor (ths): Venkateswara, Hemanth
- Thesis advisor (ths): McDaniel, Troy
- Committee member: Michael, Katina
- Publisher (pbl): Arizona State University