Outlier-Aware Applications in High-Dimensional Industrial Systems
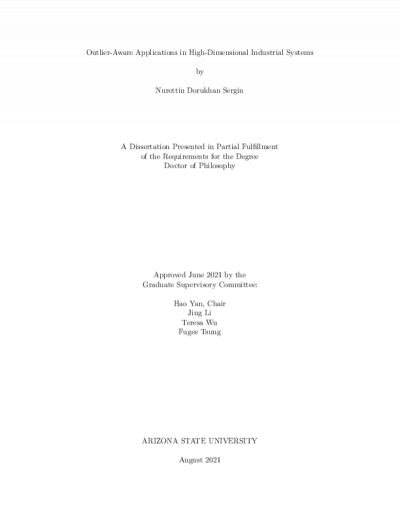
Description
High-dimensional data is omnipresent in modern industrial systems. An imaging sensor in a manufacturing plant a can take images of millions of pixels or a sensor may collect months of data at very granular time steps. Dimensionality reduction techniques are commonly used for dealing with such data. In addition, outliers typically exist in such data, which may be of direct or indirect interest given the nature of the problem that is being solved. Current research does not address the interdependent nature of dimensionality reduction and outliers. Some works ignore the existence of outliers altogether—which discredits the robustness of these methods in real life—while others provide suboptimal, often band-aid solutions. In this dissertation, I propose novel methods to achieve outlier-awareness in various dimensionality reduction methods. The problem is considered from many different angles depend- ing on the dimensionality reduction technique used (e.g., deep autoencoder, tensors), the nature of the application (e.g., manufacturing, transportation) and the outlier structure (e.g., sparse point anomalies, novelties).
Date Created
The date the item was original created (prior to any relationship with the ASU Digital Repositories.)
2021
Agent
- Author (aut): Sergin, Nurettin Dorukhan
- Thesis advisor (ths): Yan, Hao
- Committee member: Li, Jing
- Committee member: Wu, Teresa
- Committee member: Tsung, Fugee
- Publisher (pbl): Arizona State University