Influence of Goal Alignment on Delegation Decisions to Human and Automated Collaborators
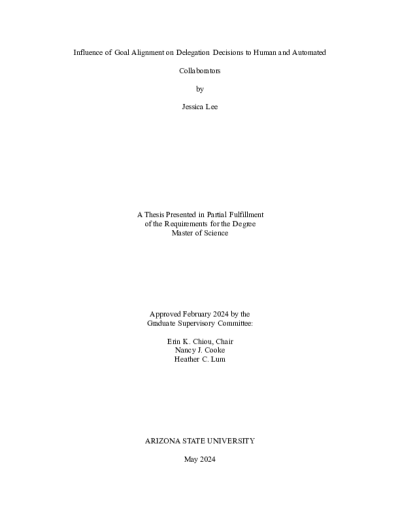
Description
Automation is becoming more autonomous, and the application of automation as a collaborator continues to be explored. A major restriction to automation’s application as a collaborator is that people often hold inaccurate expectations of their automated collaborator. Goal alignment has been shown to be beneficial in collaborations and delegation decisions among human-human and human-automation collaborations. Few studies have investigated the difference that goal alignment has on human collaborators compared to automated collaborators. In this 2 (goal aligned or misaligned) x 2 (human or automated) between-subjects study, participants complete a simplified triage patient task and then are given the opportunity to stay with their manual task solution or to delegate their decision and go with their collaborator’s recommendation. Participants never delegated to collaborators with goals that were not aligned to theirs. Participants working with human collaborators that have similar goals to them were more often delegated to and more often associated with a better triage performance. These results can inform the design of similar systems that foster collaboration and achieve better team performance. Although goal alignment was crucial for delegation decisions, it was difficult to achieve complete agreement of goals. Future research should investigate effective methods to better communicate goals among collaborators.
Date Created
The date the item was original created (prior to any relationship with the ASU Digital Repositories.)
2024
Agent
- Author (aut): Lee, Jessica Rose
- Thesis advisor (ths): Chiou, Erin K
- Committee member: Cooke, Nancy J
- Committee member: Lum, Heather C
- Publisher (pbl): Arizona State University