Data-driven Locational Marginal Price Prediction From Market Participants’ Perspective
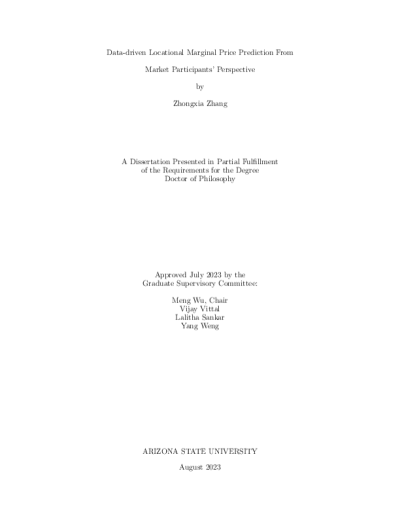
Description
Energy market participants' optimal bidding strategies and profit maximization is built upon accurate locational marginal price (LMP) predictions. In wholesale electricity markets, LMPs are strongly spatio-temporal correlated. Without access to confidential information on system topology, model parameters, or market operation conditions, market participants can only accept data-driven methods to utilize publicly available market data to predict LMPs. Most previous data-driven studies on LMP forecasting only leveraged temporal correlations among historical LMPs, and very few of them learned the spatial correlations to improve forecasting accuracy. In this dissertation, unsupervised data-driven approaches are proposed to predict LMPs in real-world energy markets from market participants' perspective. To take advantage of the spatio-temporal correlations, a general data structure is introduced to organize system-wide heterogeneous market data streams into the format of market data 2-dimensional (2D) arrays and 3-dimensional (3D) tensors. The system-wide LMP prediction problem is formulated as a sequence prediction problem. A generative adversarial network (GAN) based prediction model is adopted to learn the spatio-temporal correlations among historical LMPs preserved in the market data 3D tensors, then predict future system-wide LMPs. Multi-loss functions are introduced to assist the adversarial training procedure. A convolutional long-short-term memory (CLSTM)-based GAN is developed to improve forecasting accuracy. All LMP price components are jointly determined by the interactions between the market clearing process and the generator bidding process. The market participants’ LMP forecasting problem can be formulated as a sequential decision-making model considering the interactive market clearing and generation bidding decision-making processes. The spatio-temporal decision transformer is proposed to learn the underlying sequential decision-making model from historical spatio-temporal market data and forecast LMPs as the future actions of these interactive decision-making processes. A two-stage approach is proposed to incorporate historical generation bids into energy price prediction from market participants' perspective. Historical generation bids are taken as the first stage's output and the second stage's input in the training process. The implicit correlation among locational bids, demands, and energy prices is learned to improve price forecasting accuracy. The proposed approaches are verified through case studies using both real-world and simulated data.
Date Created
The date the item was original created (prior to any relationship with the ASU Digital Repositories.)
2023
Agent
- Author (aut): ZHANG, ZHONGXIA
- Thesis advisor (ths): Wu, Meng MW
- Committee member: Vittal, Vijay VV
- Committee member: Sankar, Lalitha LS
- Committee member: Weng, Yang YW
- Publisher (pbl): Arizona State University