Agora: Introducing the Internet’s Opinion to Traditional Stock Analysis and Prediction
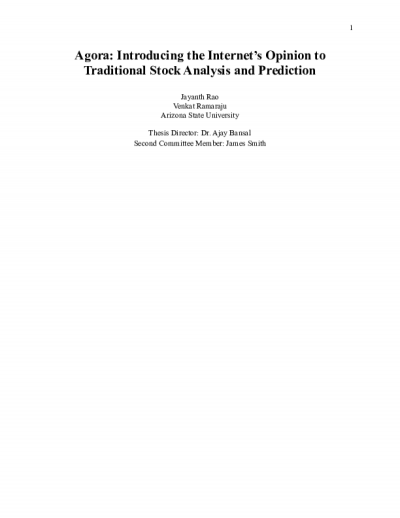
This project aims to incorporate the aspect of sentiment analysis into traditional stock analysis to enhance stock rating predictions by applying a reliance on the opinion of various stocks from the Internet. Headlines from eight major news publications and conversations from Yahoo! Finance’s “Conversations” feature were parsed through the Valence Aware Dictionary for Sentiment Reasoning (VADER) natural language processing package to determine numerical polarities which represented positivity or negativity for a given stock ticker. These generated polarities were paired with stock metrics typically observed by stock analysts as the feature set for a Logistic Regression machine learning model. The model was trained on roughly 1500 major stocks to determine a binary classification between a “Buy” or “Not Buy” rating for each stock, and the results of the model were inserted into the back-end of the Agora Web UI which emulates search engine behavior specifically for stocks found in NYSE and NASDAQ. The model reported an accuracy of 82.5% and for most major stocks, the model’s prediction correlated with stock analysts’ ratings. Given the volatility of the stock market and the propensity for hive-mind behavior in online forums, the performance of the Logistic Regression model would benefit from incorporating historical stock data and more sources of opinion to balance any subjectivity in the model.
- Author (aut): Rao, Jayanth
- Co-author: Ramaraju, Venkat
- Thesis director: Bansal, Ajay
- Committee member: Smith, James
- Contributor (ctb): Barrett, The Honors College
- Contributor (ctb): Computer Science and Engineering Program
- Contributor (ctb): School of Mathematical and Statistical Sciences