Language Image Transformer
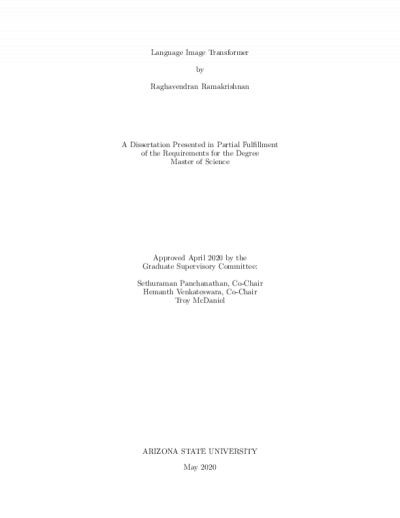
Description
Humans perceive the environment using multiple modalities like vision, speech (language), touch, taste, and smell. The knowledge obtained from one modality usually complements the other. Learning through several modalities helps in constructing an accurate model of the environment. Most of the current vision and language models are modality-specific and, in many cases, extensively use deep-learning based attention mechanisms for learning powerful representations. This work discusses the role of attention in associating vision and language for generating shared representation. Language Image Transformer (LIT) is proposed for learning multi-modal representations of the environment. It uses a training objective based on Contrastive Predictive Coding (CPC) to maximize the Mutual Information (MI) between the visual and linguistic representations. It learns the relationship between the modalities using the proposed cross-modal attention layers. It is trained and evaluated using captioning datasets, MS COCO, and Conceptual Captions. The results and the analysis offers a perspective on the use of Mutual Information Maximisation (MIM) for generating generalizable representations across multiple modalities.
Date Created
The date the item was original created (prior to any relationship with the ASU Digital Repositories.)
2020
Agent
- Author (aut): Ramakrishnan, Raghavendran
- Thesis advisor (ths): Panchanathan, Sethuraman
- Thesis advisor (ths): Venkateswara, Hemanth Kumar
- Committee member: McDaniel, Troy
- Publisher (pbl): Arizona State University