Multimodal Representation Learning for Visual Reasoning and Text-to-Image Translation
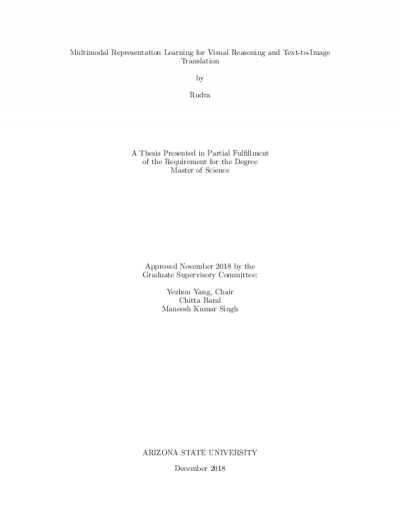
Description
Multimodal Representation Learning is a multi-disciplinary research field which aims to integrate information from multiple communicative modalities in a meaningful manner to help solve some downstream task. These modalities can be visual, acoustic, linguistic, haptic etc. The interpretation of ’meaningful integration of information from different modalities’ remains modality and task dependent. The downstream task can range from understanding one modality in the presence of information from other modalities, to that of translating input from one modality to another. In this thesis the utility of multimodal representation learning for understanding one modality vis-à-vis Image Understanding for Visual Reasoning given corresponding information in other modalities, as well as translating from one modality to the other, specifically, Text to Image Translation was investigated.
Visual Reasoning has been an active area of research in computer vision. It encompasses advanced image processing and artificial intelligence techniques to locate, characterize and recognize objects, regions and their attributes in the image in order to comprehend the image itself. One way of building a visual reasoning system is to ask the system to answer questions about the image that requires attribute identification, counting, comparison, multi-step attention, and reasoning. An intelligent system is thought to have a proper grasp of the image if it can answer said questions correctly and provide a valid reasoning for the given answers. In this work how a system can be built by learning a multimodal representation between the stated image and the questions was investigated. Also, how background knowledge, specifically scene-graph information, if available, can be incorporated into existing image understanding models was demonstrated.
Multimodal learning provides an intuitive way of learning a joint representation between different modalities. Such a joint representation can be used to translate from one modality to the other. It also gives way to learning a shared representation between these varied modalities and allows to provide meaning to what this shared representation should capture. In this work, using the surrogate task of text to image translation, neural network based architectures to learn a shared representation between these two modalities was investigated. Also, the ability that such a shared representation is capable of capturing parts of different modalities that are equivalent in some sense is proposed. Specifically, given an image and a semantic description of certain objects present in the image, a shared representation between the text and the image modality capable of capturing parts of the image being mentioned in the text was demonstrated. Such a capability was showcased on a publicly available dataset.
Visual Reasoning has been an active area of research in computer vision. It encompasses advanced image processing and artificial intelligence techniques to locate, characterize and recognize objects, regions and their attributes in the image in order to comprehend the image itself. One way of building a visual reasoning system is to ask the system to answer questions about the image that requires attribute identification, counting, comparison, multi-step attention, and reasoning. An intelligent system is thought to have a proper grasp of the image if it can answer said questions correctly and provide a valid reasoning for the given answers. In this work how a system can be built by learning a multimodal representation between the stated image and the questions was investigated. Also, how background knowledge, specifically scene-graph information, if available, can be incorporated into existing image understanding models was demonstrated.
Multimodal learning provides an intuitive way of learning a joint representation between different modalities. Such a joint representation can be used to translate from one modality to the other. It also gives way to learning a shared representation between these varied modalities and allows to provide meaning to what this shared representation should capture. In this work, using the surrogate task of text to image translation, neural network based architectures to learn a shared representation between these two modalities was investigated. Also, the ability that such a shared representation is capable of capturing parts of different modalities that are equivalent in some sense is proposed. Specifically, given an image and a semantic description of certain objects present in the image, a shared representation between the text and the image modality capable of capturing parts of the image being mentioned in the text was demonstrated. Such a capability was showcased on a publicly available dataset.
Date Created
The date the item was original created (prior to any relationship with the ASU Digital Repositories.)
2018
Agent
- Author (aut): Saha, Rudra
- Thesis advisor (ths): Yang, Yezhou
- Committee member: Singh, Maneesh Kumar
- Committee member: Baral, Chitta
- Publisher (pbl): Arizona State University