Bayesian Network Approach to Assessing System Reliability for Improving System Design and Optimizing System Maintenance
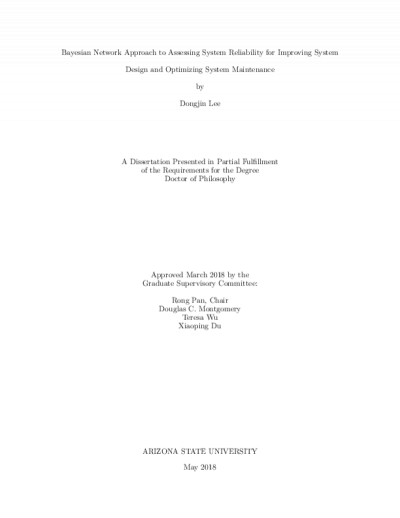
Description
A quantitative analysis of a system that has a complex reliability structure always involves considerable challenges. This dissertation mainly addresses uncertainty in- herent in complicated reliability structures that may cause unexpected and undesired results.
The reliability structure uncertainty cannot be handled by the traditional relia- bility analysis tools such as Fault Tree and Reliability Block Diagram due to their deterministic Boolean logic. Therefore, I employ Bayesian network that provides a flexible modeling method for building a multivariate distribution. By representing a system reliability structure as a joint distribution, the uncertainty and correlations existing between system’s elements can effectively be modeled in a probabilistic man- ner. This dissertation focuses on analyzing system reliability for the entire system life cycle, particularly, production stage and early design stages.
In production stage, the research investigates a system that is continuously mon- itored by on-board sensors. With modeling the complex reliability structure by Bayesian network integrated with various stochastic processes, I propose several methodologies that evaluate system reliability on real-time basis and optimize main- tenance schedules.
In early design stages, the research aims to predict system reliability based on the current system design and to improve the design if necessary. The three main challenges in this research are: 1) the lack of field failure data, 2) the complex reliability structure and 3) how to effectively improve the design. To tackle the difficulties, I present several modeling approaches using Bayesian inference and nonparametric Bayesian network where the system is explicitly analyzed through the sensitivity analysis. In addition, this modeling approach is enhanced by incorporating a temporal dimension. However, the nonparametric Bayesian network approach generally accompanies with high computational efforts, especially, when a complex and large system is modeled. To alleviate this computational burden, I also suggest to building a surrogate model with quantile regression.
In summary, this dissertation studies and explores the use of Bayesian network in analyzing complex systems. All proposed methodologies are demonstrated by case studies.
The reliability structure uncertainty cannot be handled by the traditional relia- bility analysis tools such as Fault Tree and Reliability Block Diagram due to their deterministic Boolean logic. Therefore, I employ Bayesian network that provides a flexible modeling method for building a multivariate distribution. By representing a system reliability structure as a joint distribution, the uncertainty and correlations existing between system’s elements can effectively be modeled in a probabilistic man- ner. This dissertation focuses on analyzing system reliability for the entire system life cycle, particularly, production stage and early design stages.
In production stage, the research investigates a system that is continuously mon- itored by on-board sensors. With modeling the complex reliability structure by Bayesian network integrated with various stochastic processes, I propose several methodologies that evaluate system reliability on real-time basis and optimize main- tenance schedules.
In early design stages, the research aims to predict system reliability based on the current system design and to improve the design if necessary. The three main challenges in this research are: 1) the lack of field failure data, 2) the complex reliability structure and 3) how to effectively improve the design. To tackle the difficulties, I present several modeling approaches using Bayesian inference and nonparametric Bayesian network where the system is explicitly analyzed through the sensitivity analysis. In addition, this modeling approach is enhanced by incorporating a temporal dimension. However, the nonparametric Bayesian network approach generally accompanies with high computational efforts, especially, when a complex and large system is modeled. To alleviate this computational burden, I also suggest to building a surrogate model with quantile regression.
In summary, this dissertation studies and explores the use of Bayesian network in analyzing complex systems. All proposed methodologies are demonstrated by case studies.
Date Created
The date the item was original created (prior to any relationship with the ASU Digital Repositories.)
2018
Agent
- Author (aut): Lee, Dongjin
- Thesis advisor (ths): Pan, Rong
- Committee member: Montgomery, Douglas C.
- Committee member: Wu, Teresa
- Committee member: Du, Xiaoping
- Publisher (pbl): Arizona State University