Quantitative modeling methods for analyzing clinical to public health problems
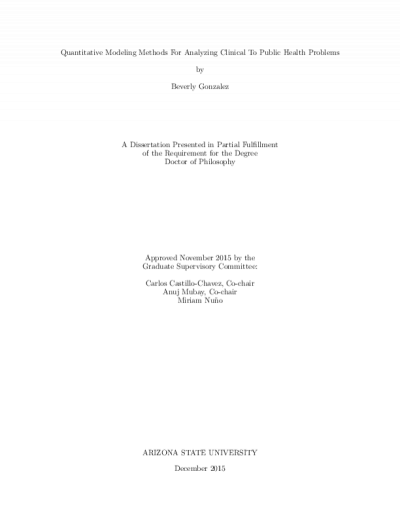
Description
Statistical Methods have been widely used in understanding factors for clinical and public health data. Statistical hypotheses are procedures for testing pre-stated hypotheses. The development and properties of these procedures as well as their performance are based upon certain assumptions. Desirable properties of statistical tests are to maintain validity and to perform well even if these assumptions are not met. A statistical test that maintains such desirable properties is called robust. Mathematical models are typically mechanistic framework, used to study dynamic interactions between components (mechanisms) of a system, and how these interactions give rise to the changes in behavior (patterns) of the system as a whole over time.
In this thesis, I have developed a study that uses novel techniques to link robust statistical tests and mathematical modeling methods guided by limited data from developed and developing regions in order to address pressing clinical and epidemiological questions of interest. The procedure in this study consists of three primary steps, namely, data collection, uncertainty quantification in data, and linking dynamic model to collected data.
The first part of the study focuses on designing, collecting, and summarizing empirical data from the only national survey of hospitals ever conducted regarding patient controlled analgesia (PCA) practices among 168 hospitals across 40 states, in order to assess risks before putting patients on PCA. I used statistical relational models and exploratory data analysis to address the question. Risk factors assessed indicate a great concern for the safety of patients from one healthcare institution to other.
In the second part, I quantify uncertainty associated with data obtained from James A Lovell Federal Healthcare Center to primarily study the effect of Benign Prostatic Hypertrophy (BPH) on sleep architecture in patients with Obstructive Sleep Apnea (OSA). Patients with OSA and BPH demonstrated significant difference in their sleep architecture in comparison to patients without BPH. One of the ways to validate these differences in sleep architecture between the two groups may be to carry out a similar study that evaluates the effect of some other chronic disease on sleep architecture in patients with OSA.
Additionally, I also address theoretical statistical questions such as (1) how to estimate the distribution of a variable in order to retest null hypothesis when the sample size is limited, and (2) how changes on assumptions (like monotonicity and nonlinearity) translate into the effect of the independent variable on the outcome variable. To address these questions we use multiple techniques such as Partial Rank Correlation Coefficients (PRCC) based sensitivity analysis, Fractional Polynomials, and statistical relational models.
In the third part, my goal was to identify socio-economic-environment-related risk factors for Visceral Leishmaniasis (VL) and use the identified critical factors to develop a mathematical model to understand VL transmission dynamics when data is highly underreported. I primarily studied the role of age-specific- susceptibility and epidemiological quantities on the dynamics of VL in the Indian state of Bihar. Statistical results provided ideas on the choice of the modeling framework and estimates of model parameters.
In the conclusion, this study addressed three primary theoretical modeling-related questions (1) how to analyze collected data when sample size limited, and how modeling assumptions varies results of data analysis? (2) Is it possible to identify hidden associations and nonlinearity of these associations using such underpowered data and (3) how statistical models provide more reasonable structure to mathematical modeling framework that can be used in turn to understand dynamics of the system.
In this thesis, I have developed a study that uses novel techniques to link robust statistical tests and mathematical modeling methods guided by limited data from developed and developing regions in order to address pressing clinical and epidemiological questions of interest. The procedure in this study consists of three primary steps, namely, data collection, uncertainty quantification in data, and linking dynamic model to collected data.
The first part of the study focuses on designing, collecting, and summarizing empirical data from the only national survey of hospitals ever conducted regarding patient controlled analgesia (PCA) practices among 168 hospitals across 40 states, in order to assess risks before putting patients on PCA. I used statistical relational models and exploratory data analysis to address the question. Risk factors assessed indicate a great concern for the safety of patients from one healthcare institution to other.
In the second part, I quantify uncertainty associated with data obtained from James A Lovell Federal Healthcare Center to primarily study the effect of Benign Prostatic Hypertrophy (BPH) on sleep architecture in patients with Obstructive Sleep Apnea (OSA). Patients with OSA and BPH demonstrated significant difference in their sleep architecture in comparison to patients without BPH. One of the ways to validate these differences in sleep architecture between the two groups may be to carry out a similar study that evaluates the effect of some other chronic disease on sleep architecture in patients with OSA.
Additionally, I also address theoretical statistical questions such as (1) how to estimate the distribution of a variable in order to retest null hypothesis when the sample size is limited, and (2) how changes on assumptions (like monotonicity and nonlinearity) translate into the effect of the independent variable on the outcome variable. To address these questions we use multiple techniques such as Partial Rank Correlation Coefficients (PRCC) based sensitivity analysis, Fractional Polynomials, and statistical relational models.
In the third part, my goal was to identify socio-economic-environment-related risk factors for Visceral Leishmaniasis (VL) and use the identified critical factors to develop a mathematical model to understand VL transmission dynamics when data is highly underreported. I primarily studied the role of age-specific- susceptibility and epidemiological quantities on the dynamics of VL in the Indian state of Bihar. Statistical results provided ideas on the choice of the modeling framework and estimates of model parameters.
In the conclusion, this study addressed three primary theoretical modeling-related questions (1) how to analyze collected data when sample size limited, and how modeling assumptions varies results of data analysis? (2) Is it possible to identify hidden associations and nonlinearity of these associations using such underpowered data and (3) how statistical models provide more reasonable structure to mathematical modeling framework that can be used in turn to understand dynamics of the system.
Date Created
The date the item was original created (prior to any relationship with the ASU Digital Repositories.)
2015
Agent
- Author (aut): Gonzalez, Beverly, 1980-
- Thesis advisor (ths): Castillo-Chavez, Carlos
- Thesis advisor (ths): Mubayi, Anuj
- Committee member: Nuno, Miriam
- Publisher (pbl): Arizona State University