Learning with attributed networks: algorithms and applications
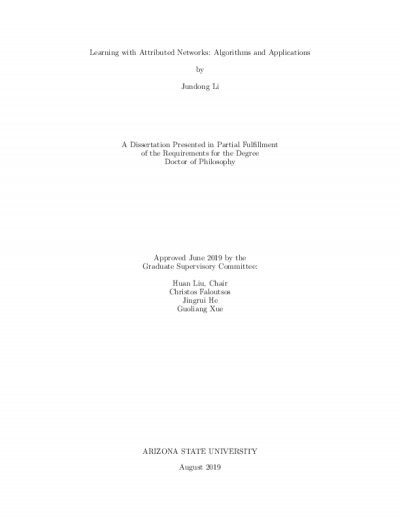
Description
Attributes - that delineating the properties of data, and connections - that describing the dependencies of data, are two essential components to characterize most real-world phenomena. The synergy between these two principal elements renders a unique data representation - the attributed networks. In many cases, people are inundated with vast amounts of data that can be structured into attributed networks, and their use has been attractive to researchers and practitioners in different disciplines. For example, in social media, users interact with each other and also post personalized content; in scientific collaboration, researchers cooperate and are distinct from peers by their unique research interests; in complex diseases studies, rich gene expression complements to the gene-regulatory networks. Clearly, attributed networks are ubiquitous and form a critical component of modern information infrastructure. To gain deep insights from such networks, it requires a fundamental understanding of their unique characteristics and be aware of the related computational challenges.
My dissertation research aims to develop a suite of novel learning algorithms to understand, characterize, and gain actionable insights from attributed networks, to benefit high-impact real-world applications. In the first part of this dissertation, I mainly focus on developing learning algorithms for attributed networks in a static environment at two different levels: (i) attribute level - by designing feature selection algorithms to find high-quality features that are tightly correlated with the network topology; and (ii) node level - by presenting network embedding algorithms to learn discriminative node embeddings by preserving node proximity w.r.t. network topology structure and node attribute similarity. As changes are essential components of attributed networks and the results of learning algorithms will become stale over time, in the second part of this dissertation, I propose a family of online algorithms for attributed networks in a dynamic environment to continuously update the learning results on the fly. In fact, developing application-aware learning algorithms is more desired with a clear understanding of the application domains and their unique intents. As such, in the third part of this dissertation, I am also committed to advancing real-world applications on attributed networks by incorporating the objectives of external tasks into the learning process.
My dissertation research aims to develop a suite of novel learning algorithms to understand, characterize, and gain actionable insights from attributed networks, to benefit high-impact real-world applications. In the first part of this dissertation, I mainly focus on developing learning algorithms for attributed networks in a static environment at two different levels: (i) attribute level - by designing feature selection algorithms to find high-quality features that are tightly correlated with the network topology; and (ii) node level - by presenting network embedding algorithms to learn discriminative node embeddings by preserving node proximity w.r.t. network topology structure and node attribute similarity. As changes are essential components of attributed networks and the results of learning algorithms will become stale over time, in the second part of this dissertation, I propose a family of online algorithms for attributed networks in a dynamic environment to continuously update the learning results on the fly. In fact, developing application-aware learning algorithms is more desired with a clear understanding of the application domains and their unique intents. As such, in the third part of this dissertation, I am also committed to advancing real-world applications on attributed networks by incorporating the objectives of external tasks into the learning process.
Date Created
The date the item was original created (prior to any relationship with the ASU Digital Repositories.)
2019
Agent
- Author (aut): Li, Jundong
- Thesis advisor (ths): Liu, Huan
- Committee member: Faloutsos, Christos
- Committee member: He, Jingrui
- Committee member: Xue, Guoliang
- Publisher (pbl): Arizona State University