Large-scale matrix completion using orthogonal rank-one matrix pursuit, divide-factor-combine, and apache spark
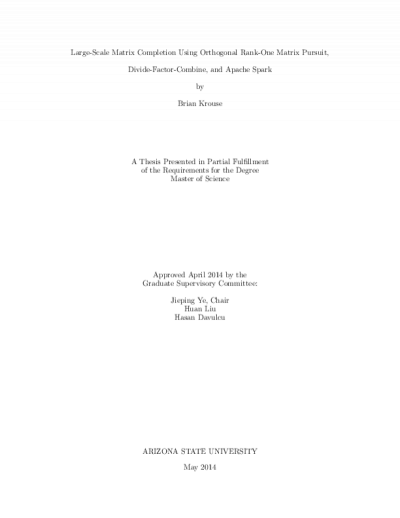
Description
As the size and scope of valuable datasets has exploded across many industries and fields of research in recent years, an increasingly diverse audience has sought out effective tools for their large-scale data analytics needs. Over this period, machine learning researchers have also been very prolific in designing improved algorithms which are capable of finding the hidden structure within these datasets. As consumers of popular Big Data frameworks have sought to apply and benefit from these improved learning algorithms, the problems encountered with the frameworks have motivated a new generation of Big Data tools to address the shortcomings of the previous generation. One important example of this is the improved performance in the newer tools with the large class of machine learning algorithms which are highly iterative in nature. In this thesis project, I set about to implement a low-rank matrix completion algorithm (as an example of a highly iterative algorithm) within a popular Big Data framework, and to evaluate its performance processing the Netflix Prize dataset. I begin by describing several approaches which I attempted, but which did not perform adequately. These include an implementation of the Singular Value Thresholding (SVT) algorithm within the Apache Mahout framework, which runs on top of the Apache Hadoop MapReduce engine. I then describe an approach which uses the Divide-Factor-Combine (DFC) algorithmic framework to parallelize the state-of-the-art low-rank completion algorithm Orthogoal Rank-One Matrix Pursuit (OR1MP) within the Apache Spark engine. I describe the results of a series of tests running this implementation with the Netflix dataset on clusters of various sizes, with various degrees of parallelism. For these experiments, I utilized the Amazon Elastic Compute Cloud (EC2) web service. In the final analysis, I conclude that the Spark DFC + OR1MP implementation does indeed produce competitive results, in both accuracy and performance. In particular, the Spark implementation performs nearly as well as the MATLAB implementation of OR1MP without any parallelism, and improves performance to a significant degree as the parallelism increases. In addition, the experience demonstrates how Spark's flexible programming model makes it straightforward to implement this parallel and iterative machine learning algorithm.
Date Created
The date the item was original created (prior to any relationship with the ASU Digital Repositories.)
2014
Agent
- Author (aut): Krouse, Brian
- Thesis advisor (ths): Ye, Jieping
- Committee member: Liu, Huan
- Committee member: Davulcu, Hasan
- Publisher (pbl): Arizona State University