Reliable Distributed Management in Uncertain Environments
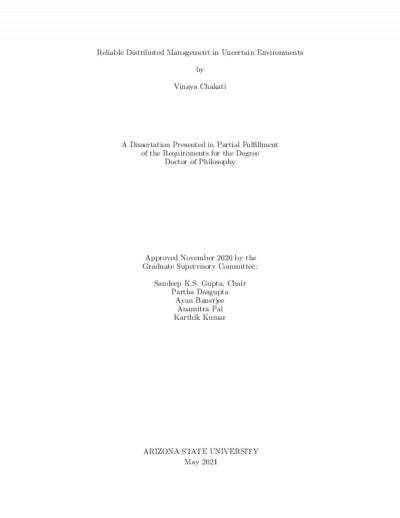
Description
Increase in the usage of Internet of Things(IoT) devices across physical systems has provided a platform for continuous data collection, real-time monitoring, and extracting useful insights. Limited computing power and constrained resources on the IoT devices has driven the physical systems to rely on external resources such as cloud computing for handling compute-intensive and data-intensive processing. Recently, physical environments have began to explore the usage of edge devices for handling complex processing. However, these environments may face many challenges suchas uncertainty of device availability, uncertainty of data relevance, and large set of geographically dispersed devices. This research proposes the design of a reliable distributed management system that focuses on the following objectives: 1. improving the success rate of task completion in uncertain environments. 2. enhancing the reliability of the applications and 3. support latency sensitive applications. Main modules of the proposed system include:
1. A novel proactive user recruitment approach to improve the success rate of the task completion.
2.Contextual data acquisition and integration of false data detection for enhancing the reliability of the applications.
3. Novel distributed management of compute resources for achieving real-time monitoring and to support highly responsive
applications.
User recruitment approaches select the devices for offloading computation. Proposed proactive user recruitment module selects an optimized set of devices that match the resource requirements of the application. Contextual data acquisition
module banks on the contextual requirements for identifying the data sources that are more useful to the application. Proposed reliable distributed management system can be used as a framework for offloading the latency sensitive applications across the volunteer computing edge devices.
Date Created
The date the item was original created (prior to any relationship with the ASU Digital Repositories.)
2021
Agent
- Author (aut): CHAKATI, VINAYA
- Thesis advisor (ths): Gupta, Sandeep K.S
- Committee member: Dasgupta, Partha
- Committee member: Banerjee, Ayan
- Committee member: Pal, Anamitra
- Committee member: Kumar, Karthik
- Publisher (pbl): Arizona State University