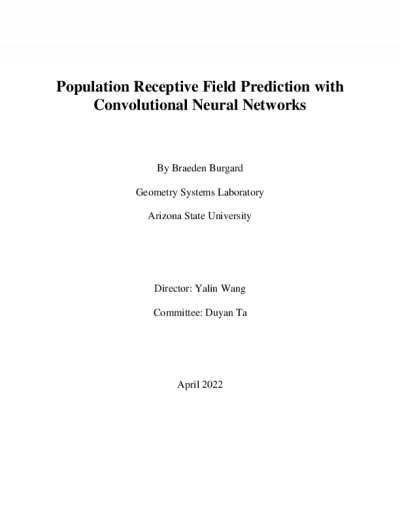
Description
The Population Receptive Field (pRF) model is widely used to predict the location (retinotopy) and size of receptive fields on the visual space. Doing so allows for the creation of a mapping from locations in the visual field to the associated groups of neurons in the cortical region (within the visual cortex of the brain). However, using the pRF model is very time consuming. Past research has focused on the creation of Convolutional Neural Networks (CNN) to mimic the pRF model in a fraction of the time, and they have worked well under highly controlled conditions. However, these models have not been thoroughly tested on real human data. This thesis focused on adapting one of these CNNs to accurately predict the retinotopy of a real human subject using a dataset from the Human Connectome Project. The results show promise towards creating a fully functioning CNN, but they also expose new challenges that must be overcome before the model could be used to predict the retinotopy of new human subjects.
Details
Title
- Population Receptive Field Prediction with Convolutional Neural Networks
Contributors
- Burgard, Braeden (Author)
- Wang, Yalin (Thesis director)
- Ta, Duyan (Committee member)
- Barrett, The Honors College (Contributor)
- School of International Letters and Cultures (Contributor)
- Computer Science and Engineering Program (Contributor)
- School of Mathematical and Statistical Sciences (Contributor)
Date Created
The date the item was original created (prior to any relationship with the ASU Digital Repositories.)
2022-05
Resource Type
Collections this item is in