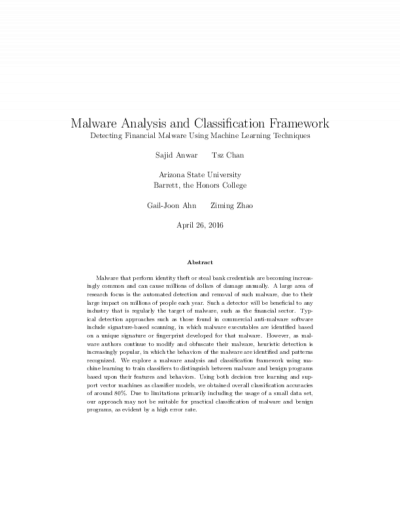
Description
Malware that perform identity theft or steal bank credentials are becoming increasingly common and can cause millions of dollars of damage annually. A large area of research focus is the automated detection and removal of such malware, due to their large impact on millions of people each year. Such a detector will be beneficial to any industry that is regularly the target of malware, such as the financial sector. Typical detection approaches such as those found in commercial anti-malware software include signature-based scanning, in which malware executables are identified based on a unique signature or fingerprint developed for that malware. However, as malware authors continue to modify and obfuscate their malware, heuristic detection is increasingly popular, in which the behaviors of the malware are identified and patterns recognized. We explore a malware analysis and classification framework using machine learning to train classifiers to distinguish between malware and benign programs based upon their features and behaviors. Using both decision tree learning and support vector machines as classifier models, we obtained overall classification accuracies of around 80%. Due to limitations primarily including the usage of a small data set, our approach may not be suitable for practical classification of malware and benign programs, as evident by a high error rate.
Details
Title
- Malware Analysis and Classification Framework: Detecting Financial Malware Using Machine Learning Techniques
Contributors
- Anwar, Sajid (Co-author)
- Chan, Tsz (Co-author)
- Ahn, Gail-Joon (Thesis director)
- Zhao, Ziming (Committee member)
- Computer Science and Engineering Program (Contributor)
- Barrett, The Honors College (Contributor)
Date Created
The date the item was original created (prior to any relationship with the ASU Digital Repositories.)
2016-05
Resource Type
Collections this item is in