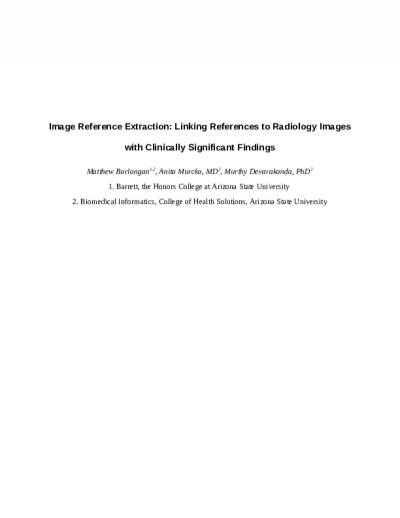
Description
Background: Pulmonary embolism is a deadly condition that is often diagnosed using a technique known as computed tomography pulmonary angiography (CTPA). CTPA reports are free-text, narrative-style forms of documentation conferring radiologist findings—both primary (regarding pulmonary embolism) and incidental. This project seeks to combine simple natural language processing (NLP) techniques, such as regular expressions and rules, to build upon and
further process output from a machine learning based named entity recognition (NER) tool for the purposes of (1) linking references to radiological images with the corresponding clinical findings and (2) extracting primary and incidental findings.
Methods: The project’s system utilized a regular expression to extract image references. All CTPA reports were first processed with NER software to obtain the text and spans of clinical findings. A heuristic was used to determine the appropriate clinical finding that should be linked with a particular image reference. Another regular expression was used to extract primary findings from NER output; the remaining findings were considered incidental. Performance was
assessed against a gold standard, which was based upon a manually annotated version of the CTPA reports used in this project.
Results: Extraction of image references achieved a 100% accuracy. Linkages between these references and exact gold standard spans of the clinical findings achieved a precision of 0.24, a recall of 0.22, and an F1 score of 0.23. Linkages with partial spans of clinical findings as determined by the gold standard achieved a precision of 0.71, a recall of 0.67, and an F1 score of 0.69. Primary and incidental finding extraction achieved a precision of 0.67, a recall of 0.80, and
an F1 score of 0.73.
Discussion: Various elements reduced system performance such as the difficulty of exactly matching the spans of clinical findings from NER output with those found in the gold standard. The heuristic linking clinical findings and image references was especially sensitive to NER false positives and false negatives due to its assumption that the appropriate clinical finding was that which was immediately prior to the image reference. Although the system did not perform as well as hoped, lessons were learned such as the need for clear research methodology and proper gold standard creation; without a proper gold standard, problem scope and system performance cannot be properly assessed. Improvements to the system include creating a more robust heuristic, sifting NER false positives, and training the NER tool used on a dataset of CTPA reports.
further process output from a machine learning based named entity recognition (NER) tool for the purposes of (1) linking references to radiological images with the corresponding clinical findings and (2) extracting primary and incidental findings.
Methods: The project’s system utilized a regular expression to extract image references. All CTPA reports were first processed with NER software to obtain the text and spans of clinical findings. A heuristic was used to determine the appropriate clinical finding that should be linked with a particular image reference. Another regular expression was used to extract primary findings from NER output; the remaining findings were considered incidental. Performance was
assessed against a gold standard, which was based upon a manually annotated version of the CTPA reports used in this project.
Results: Extraction of image references achieved a 100% accuracy. Linkages between these references and exact gold standard spans of the clinical findings achieved a precision of 0.24, a recall of 0.22, and an F1 score of 0.23. Linkages with partial spans of clinical findings as determined by the gold standard achieved a precision of 0.71, a recall of 0.67, and an F1 score of 0.69. Primary and incidental finding extraction achieved a precision of 0.67, a recall of 0.80, and
an F1 score of 0.73.
Discussion: Various elements reduced system performance such as the difficulty of exactly matching the spans of clinical findings from NER output with those found in the gold standard. The heuristic linking clinical findings and image references was especially sensitive to NER false positives and false negatives due to its assumption that the appropriate clinical finding was that which was immediately prior to the image reference. Although the system did not perform as well as hoped, lessons were learned such as the need for clear research methodology and proper gold standard creation; without a proper gold standard, problem scope and system performance cannot be properly assessed. Improvements to the system include creating a more robust heuristic, sifting NER false positives, and training the NER tool used on a dataset of CTPA reports.
Details
Title
- Image Reference Extraction: Linking References to Radiology Images with Clinically Significant Findings
Contributors
- Borlongan, Matthew Bilog (Author)
- Devarakonda, Murthy (Thesis director)
- Murcko, Anita (Committee member)
- College of Health Solutions (Contributor, Contributor)
- Barrett, The Honors College (Contributor)
Date Created
The date the item was original created (prior to any relationship with the ASU Digital Repositories.)
2019-05
Subjects
Resource Type
Collections this item is in