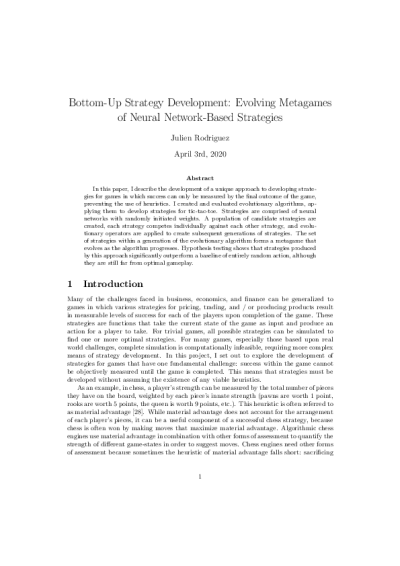
Description
In this paper, I describe the development of a unique approach to developing strategies for games in which success can only be measured by the final outcome of the game, preventing the use of heuristics. I created and evaluated evolutionary algorithms, applying them to develop strategies for tic-tac-toe. Strategies are comprised of neural networks with randomly initiated weights. A population of candidate strategies are created, each strategy competes individually against each other strategy, and evolutionary operators are applied to create subsequent generations of strategies. The set of strategies within a generation of the evolutionary algorithm forms a metagame that evolves as the algorithm progresses. Hypothesis testing shows that strategies produced by this approach significantly outperform a baseline of entirely random action, although they are still far from optimal gameplay.
Details
Title
- Bottom-Up Strategy Development: Evolving Metagames of Neural Network-Based Strategies
Contributors
- Rodriguez, Julien Guillermo (Author)
- Martin, Thomas (Thesis director)
- Powers, Brian (Committee member)
- College of Integrative Sciences and Arts (Contributor, Contributor)
- Department of Information Systems (Contributor)
- Barrett, The Honors College (Contributor)
Date Created
The date the item was original created (prior to any relationship with the ASU Digital Repositories.)
2020-05
Resource Type
Collections this item is in